Research Project Guide
How To Analyze Quantitative Data In 9 Simple Steps
Master how to analyze quantitative data with our 9 simple steps. Unlock insights and enhance your decision-making today!
Oct 23, 2024
Crunching numbers and making sense of data can feel like deciphering a secret code, especially when figuring out how to start a research project. You’ve collected all this quantitative data, but now what? Analyzing it effectively is crucial to draw meaningful conclusions, save time, and keep your writing sharp. In this guide, you'll find the essentials to conduct fast research and write efficiently, giving you a clear path to tackle your data analysis confidently.
Otio’s AI research and writing partner can help you achieve your research goals faster and more efficiently.
Table Of Contents
Quantitative Data Vs. Qualitative Data
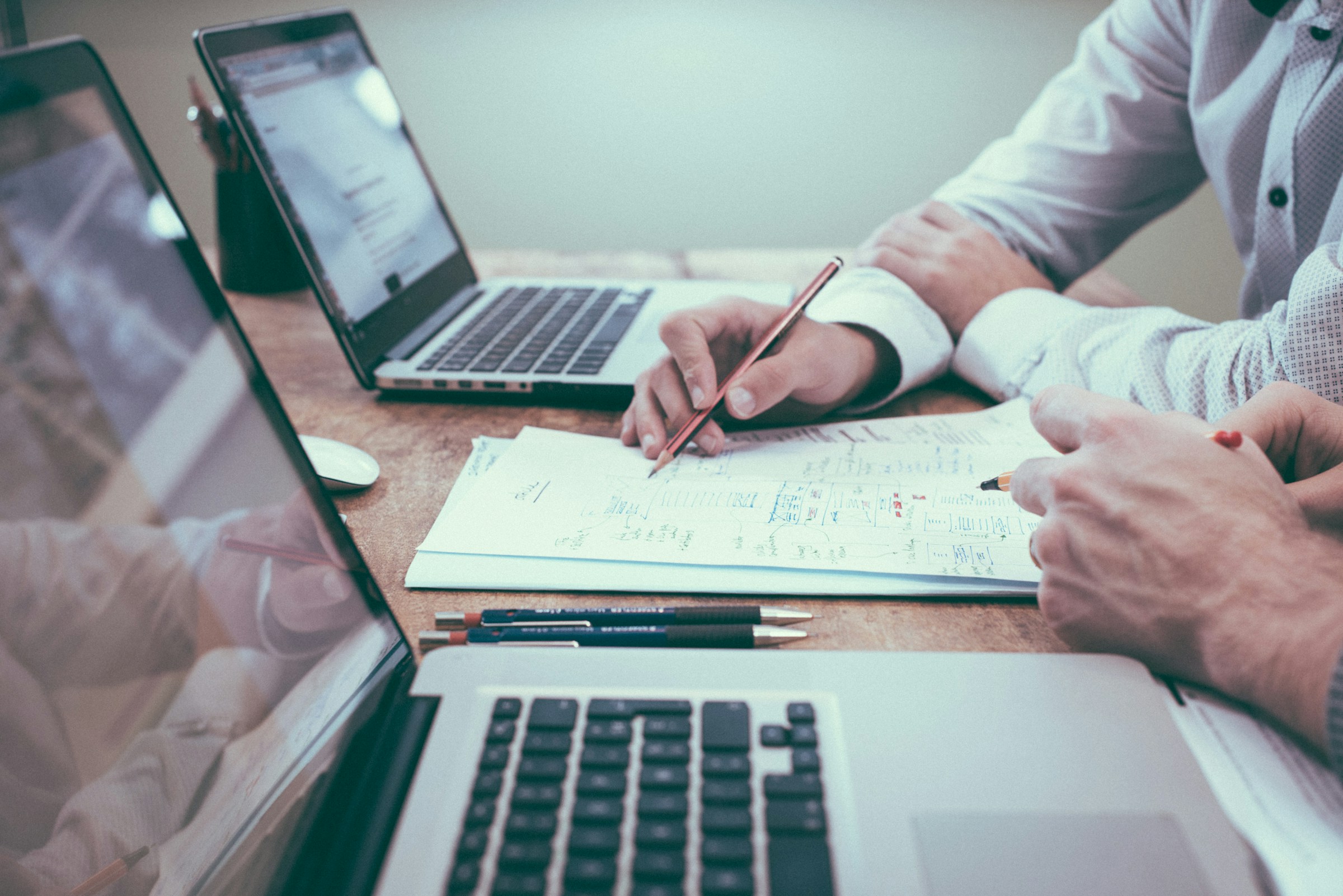
Quantitative data is all about the numbers. It involves measurements or counts, expressed in numerical terms, and deals with questions like “how many,” “how much,” or “how often.” Think of it as the stats that underpin your research. For instance, you might track how many people downloaded an app or how much a teenager grew in height over a year. It’s likely quantitative data if you can count or measure it.
On the flip side, qualitative data is all about characteristics or types. These categories or descriptions help you understand the “what” of your research. It could be the different hair colors people have or the variety of occupations represented in a study. You're dealing with qualitative data, whether a type or a kind.
When to Use Quantitative and Qualitative Data
Quantitative and qualitative data often work best together. For example, you might gather quantitative data on annual income and then collect qualitative data on occupation to understand what types of jobs have the highest earnings. This gives you a more detailed and accurate picture of the situation. You can collect both data types from the same study participant, depending on whether your variable of interest is numerical or categorical.
Identifying Data Types Before Collection
An easy way to determine which data type you’re dealing with is to determine whether your variable is numerical or categorical. The data will be quantitative if it’s numerical, like age or income. If it’s categorical, like hair color or occupation, it’s qualitative. Understanding this distinction upfront can help you plan how to collect and analyze your data.
How To Analyze Quantitative Data In 9 Simple Steps
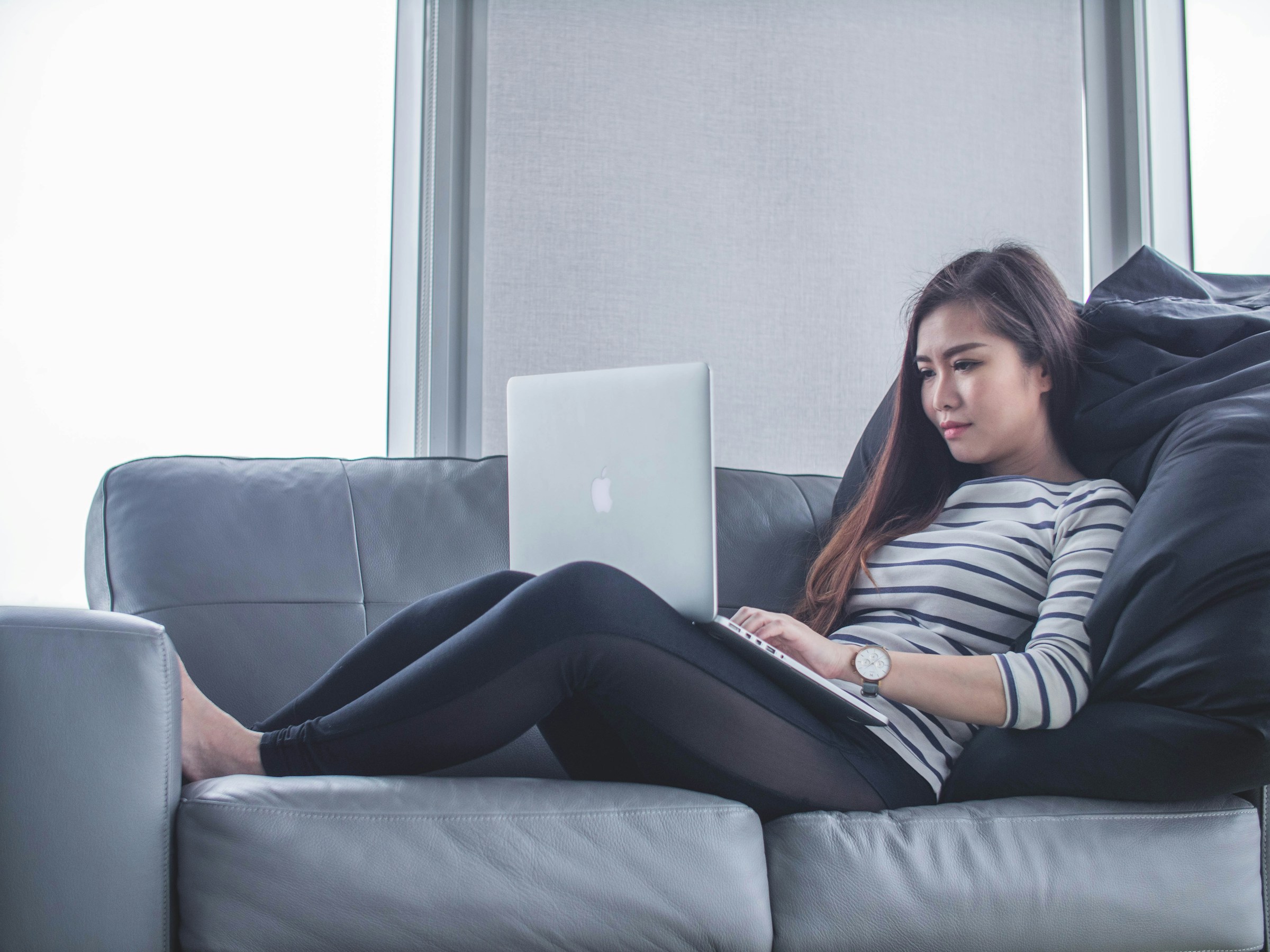
1. Kickstart Your Analysis with a Clear Problem Statement
The first step in analyzing quantitative data is pinpointing your research question. Begin by defining a clear problem statement that guides your entire study. This statement will inform your data collection and analysis methods, ensuring your efforts align with your research objectives.
2. Gather Data that Matters
Once your problem statement is set, focus on collecting relevant data. Choose an approach that best suits your research question, whether it involves surveys, experiments, or secondary data analysis. Remember to prioritize data that directly addresses your research objectives, ensuring you gather relevant and reliable information.
3. Process Your Data for Insights
When data comes in, it’s often messy and needs cleaning. Use data-cleaning techniques to remove errors, fill in missing values, and handle outliers. This stage is crucial for ensuring data quality and extracting meaningful information to drive your analysis forward.
4. Summarize with Descriptive Statistics
Once your data is clean, use descriptive statistics like mean, median, and mode to summarize it. These measures help highlight critical features of your dataset, providing a clear overview that can guide your subsequent analysis.
5. Visualize to Understand
Graphs and charts are your friends here. Use them to visually represent your data, making patterns and trends more apparent. This step is constructive for identifying relationships and anomalies that might need clarification in raw data.
6. Formulate Hypotheses for Testing
With a better understanding of your data, you can start developing hypotheses to test. These should be based on your exploratory data analysis (EDA) findings and guide your next steps in the analysis process.
7. Test Relationships with Hypothesis Testing
Now, it’s time to test your hypotheses. Use t-tests, chi-square tests, and regression analysis to explore relationships between data points. These tests will help you understand whether your identified patterns are statistically significant.
8. Evaluate Differences with Statistical Tests
To assess the significance of any differences, apply appropriate statistical tests. This step is crucial for determining whether your findings are meaningful and whether they should inform your research conclusions.
9. Conclude with Confidence
After analyzing your data, summarize your key findings and their implications. This step involves interpreting your results and explaining their significance in the context of your research question.
For those navigating the complexities of research, Otio is your AI research and writing partner, designed to streamline your workflow and help you turn insights into outcomes. Try Otio for free today!
Related Reading
• How to Find Academic Sources
• Can Ai Write a Paper for Me
• How Long Does It Take to Write a Research Paper
• How to Create a Research Question
• Research Methodology Types
• How to Organize a Research Paper
• Argumentative Essay Topics
• Methods Section of Research Paper
What Are The Best Methods of Quantitative Analysis?
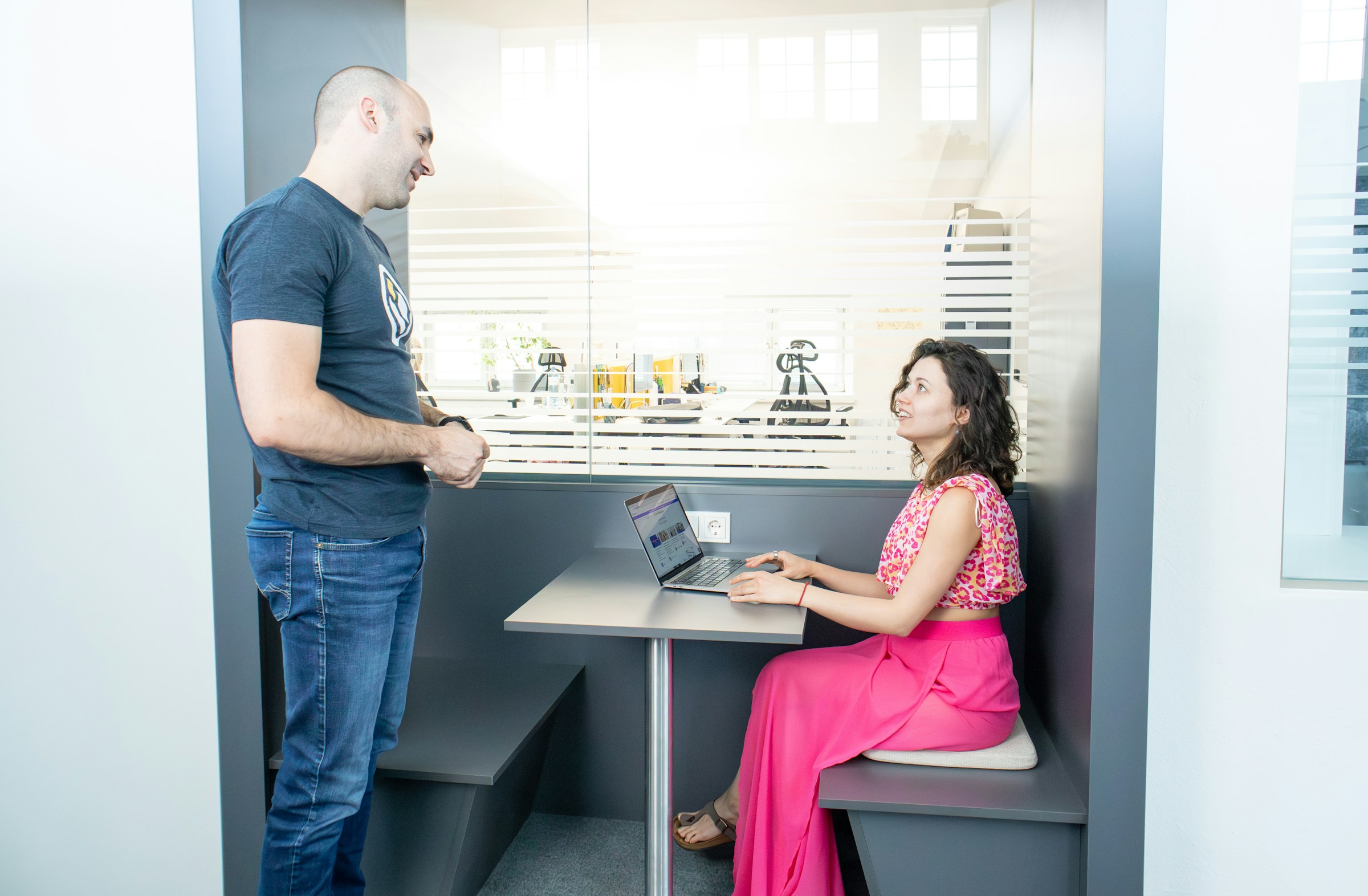
1. Use Otio to Tame Content Chaos
In today’s world, knowledge workers, researchers, and students face overwhelming content. Juggling fragmented, complex tools just isn’t cutting it anymore. Otio changes the game by offering an AI-native workspace specifically for researchers. It streamlines collecting data from bookmarks, tweets, books, and YouTube videos. But that’s not all. Otio extracts key insights with AI-generated notes and source-grounded Q&A chats.
When you're ready to create, it helps you draft outputs using your collected sources. Otio takes you faster than ever from the reading list to the first draft. Plus, it speeds up writing research papers and essays. Researchers love its AI-generated notes on bookmarks, the ability to chat with individual links or entire knowledge bases, and AI-assisted writing. Make Otio your AI research and writing partner today—try it for free!
2. Descriptive Analysis: Finding Patterns in Data
Descriptive analysis involves uncovering your dataset's patterns, trends, and critical insights. It is a way to summarize and present essential features without drawing broad conclusions about the entire dataset. This method uses the mean, mode, and median central tendency measures.
The mean is your average—sum up all your values and divide by the total number of observations. The mode is the most frequently occurring value, while the median is the middle value in a sorted dataset. Descriptive analysis is versatile and applicable in fields like robotics, fraud detection, environmental analysis, etc.
3. Correlation Analysis: Measuring Relationships
Correlation analysis evaluates the strength and direction of relationships between two or more quantitative variables. The correlation coefficient, denoted by “r,” measures how changes in one variable correspond to changes in another. Values range from -1 to 1, with positive values indicating positive correlation, negative values showing negative correlation, and zero suggesting weak or no linear correlation. Correlation analysis is used in navigation and localization to evaluate the correlation between sensor data and navigation algorithms. It’s also handy for identifying potential faults and preventing errors.
4. Regression Analysis: Predicting Outcomes
Regression analysis examines the relationship between one dependent variable and one or more independent variables. The goal is to understand how changes in the independent variables affect the dependent variable. Linear regression, for instance, draws a straight line through data points to help predict changes in one variable based on changes in another. It’s useful for facial landmark detection in computer vision, where pixel values help localize facial landmarks like eyes, nose, and mouth. Regression models help predict outcomes accurately.
5. Cluster Analysis: Grouping Similar Data
Cluster analysis groups similar data points into clusters based on certain features or characteristics. It helps identify inherent patterns or structures within your data, giving you a better understanding of relationships among the data points. In robotics, cluster analysis might group similar sensor readings from various robots, helping identify distinct behavior patterns or environmental conditions. Clustering data from robot sensors could reveal clusters representing specific tasks, allowing for more efficient and adaptive robotic decision-making.
6. Time-Series Analysis: Understanding Temporal Data
Time-series analysis focuses on studying sequential data points collected over a specific period. It helps identify patterns, trends, and structures within the data to predict observed phenomena over time. Analyzing traffic patterns with Google Maps is a great example. Time-series analysis of location data reveals trends in popular places. By analyzing temporal data, you can understand how behavior changes over time.
7. Graphical Analysis: Visualizing Complex Data
Graphical analysis visually represents data through graphs or charts, making complex information more accessible and understandable. It’s a valuable tool for drawing insights from data at a glance. Use bar charts to compare quantities among different categories, line charts to display trends over time, and scatter plots to show relationships between two continuous variables. Box plots highlight central tendency and variability, while time series plots display data in sequential order. Pie charts present proportions, and heatmaps use color gradients to represent data points.
Types of Quantitative Data
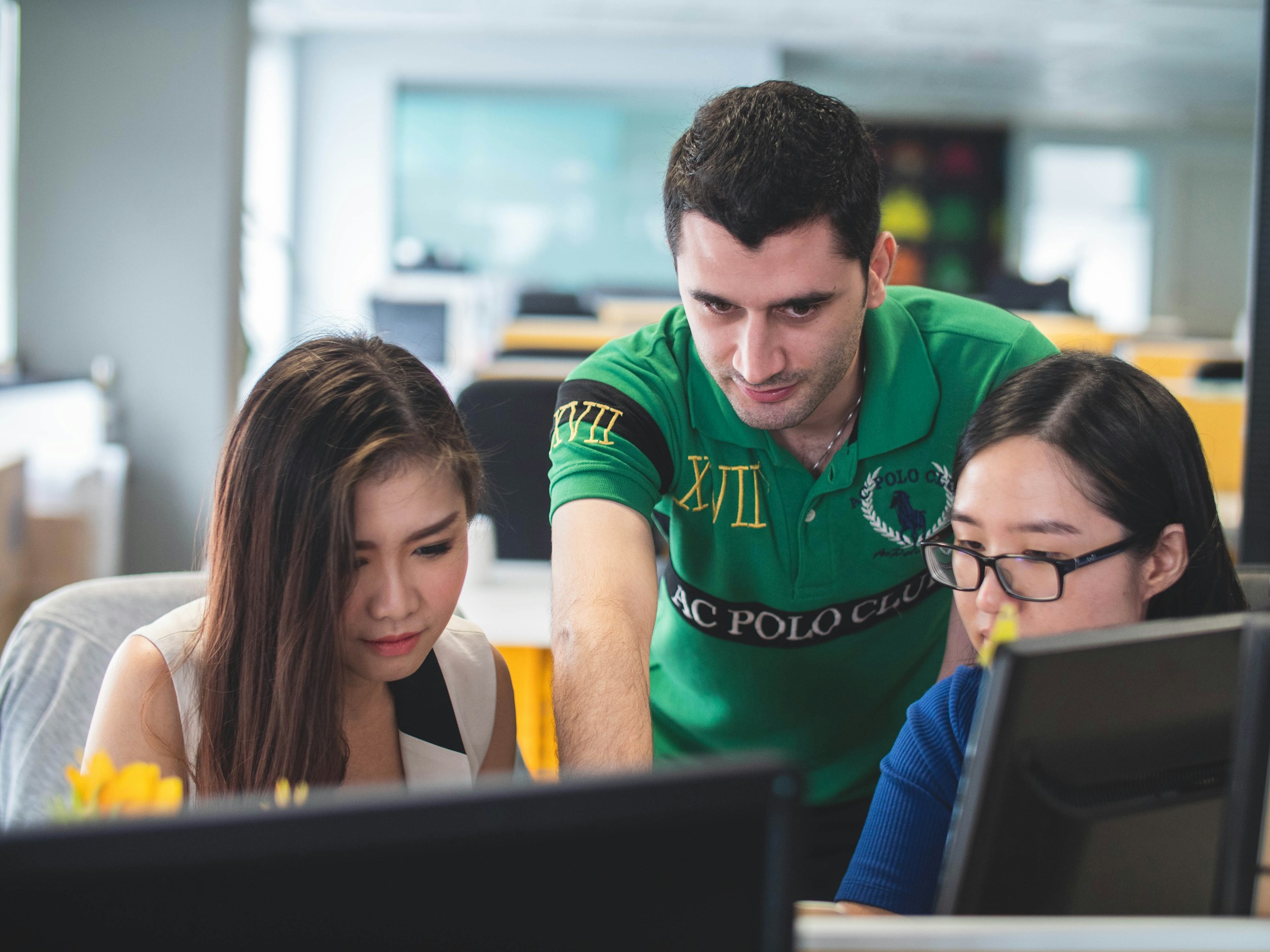
Getting to Know Discrete Data
When analyzing quantitative data, you’ll encounter discrete data of specific, countable values. Think about how you would tally up the number of students in a classroom or the points scored in a game. These values are whole numbers and don’t break down into smaller units. You can easily spot discrete data by asking if the data can be counted. This type of information is often represented in tally, bar, or pie charts.
Consider these examples: the number of players on a team, candies in a jar, exam questions, or even your shoe size. These are all classic instances of discrete data.
Understanding Continuous Data
Continuous data, on the other hand, can take any value within a range and often changes over time. For example, imagine tracking the temperature throughout the day or measuring a child’s growing height. This data is typically shown with line graphs, illustrating changes over time.
Continuous data breaks down further into ratio and interval data. Ratio data allows you to calculate geometric or harmonic means, while interval data uses arithmetic means. Examples of continuous data include daily temperatures, a baby’s height, a child’s weight, wind speeds, and the length of a leaf.
Related Reading
• How to Write a Psychology Research Paper
• Research Questions Examples
• Research Paper Abstract Example
• How to Write Results in a Research Paper
• Title Page for Research Paper
• How to Cite a Research Paper
• Best AI for Data Analysis
• How to Write a Discussion in a Research Paper
• Best AI for Writing Research Papers
• Data Collection Tools
• Ai Visualization Tools
When To Use Quantitative Analysis
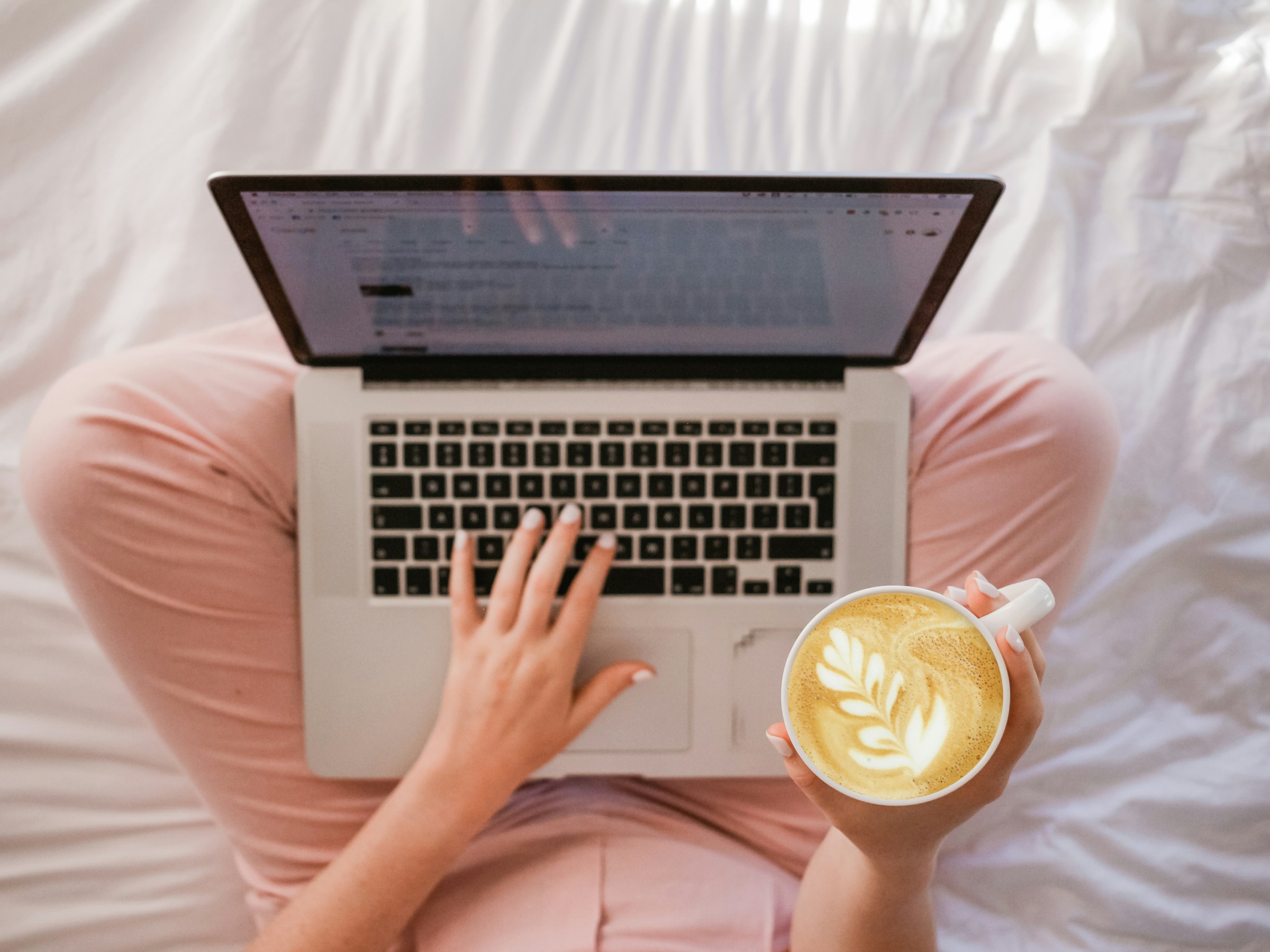
Quantitative Analysis: Understanding Group Differences
When you're in the business of figuring out how different groups stack up against each other, quantitative analysis is your go-to tool. Consider a company looking to understand which jacket colors are flying off the shelves. By surveying 1,000 customers, the company can see that black jackets are the top pick for 40% of folks. Gray comes in at 30%, with red trailing at 20%. With just a bit of number-crunching, the company knows to stock up on the popular colors and ditch the less loved ones. That’s the potential of quantitative analysis for understanding differences.
Quantitative Analysis: Exploring Relationships Between Variables
Ever wonder how two things are connected? Quantitative analysis is critical to unraveling those relationships. Take voter turnout and weather, for instance. By comparing weather data with voter turnout records from 50 cities over 20 years, researchers discovered that voter turnout drops by 5% for every 10-degree drop in temperature. That’s a big deal for understanding voter behavior and planning future campaigns. Quantitative analysis makes it easy to see how one thing influences another.
Quantitative Analysis: Testing Hypotheses with Rigor
When you’re looking to prove a hypothesis, you need a tool that can withstand scrutiny. That’s where quantitative analysis comes in. Think of a medical research team testing a new vaccine. By conducting a randomized controlled trial with 10,000 participants, they find that the vaccinated group has a significantly lower infection rate. With a 95% confidence level, they can say with certainty that the vaccine works. That’s the rigor you need to ensure your findings hold up.
Supercharge Your Researching Ability With Otio — Try Otio for Free Today
These days, we're all drowning in content. Researchers and students know this struggle well. Your inbox could be clearer, your bookmarks overflow and your read-later list should be shorter. You might have five different apps to manage it all—and if that's working for you, then you're in the minority. With AI making content creation more accessible than ever, this problem will only get worse. That's where Otio comes in. Otio is an AI-native workspace that helps researchers collect, extract takeaways, and create outputs from one place. It's a game changer for anyone who struggles to manage their research. Try Otio for free today!
What is Otio and How Does it Help Researchers?
Otio is an AI-native workspace designed to help researchers manage their research. It provides tools that allow researchers to collect, extract takeaways, and create outputs—all from one place. Here’s how it works: Otio enables you to collect data from many sources, from bookmarks, tweets, and extensive books to YouTube videos. It then extracts critical takeaways with detailed AI-generated notes and a source-grounded Q&A chat.
Finally, it helps you create draft outputs using your collected sources. You can go from the reading list to the first draft faster. Otio also enables you to write research papers/essays faster. Its top features include AI-generated notes on all bookmarks, the ability to chat with individual links or entire knowledge bases, and AI-assisted writing.
Collect and Organize Your Research with Otio
Otio makes collecting and organizing a wide range of data sources easy. Add bookmarks, tweets, and extensive books to your Otio workspace. You can also add YouTube videos and articles. Once you've added your sources, Otio organizes them in a way that makes sense for your research. You can then access your sources from one place, making it easy to find what you need when you need it.
Extract Key Takeaways with AI-generated Notes
One of Otio’s standout features is its AI-generated notes. After adding your sources, Otio analyzes them and creates detailed notes summarizing the key takeaways. These notes are grounded in the source, so you can trust they’re accurate. You can also use Otio’s Q&A chat to ask questions about your sources and get answers based on the content. This feature benefits researchers who need to understand information quickly.
Create Draft Outputs with AI-Assisted Writing
Once you’ve collected and organized your sources and extracted key takeaways, Otio helps you create draft outputs. Its AI-assisted writing feature uses the information you gather to help you write research papers and essays faster. You can use this feature to get a head start on your writing or to get unstuck when you’re having trouble getting started.
Related Reading
• Note-taking AI for Students
• Milanote vs Notion
• Obsidian vs Evernote
• Claude AI Alternative
• Milanote vs Miro
• Logseq vs Obsidian
• Best Chat Gpt Alternatives
• Zotero vs Mendeley
• Writesonic vs Jasper