AI Workflow Management
Types of AI Agents + 11 Real-Life Examples
Discover the different types of AI agents with 11 real-life examples showcasing their roles in everyday applications and industries.
Jan 22, 2025
Managing and organizing research and writing projects can be overwhelming, especially as the scope of your work grows. AI workflow management can help you stay on track and automate many tedious tasks that reduce productivity. Researching and writing an article or paper often involves collecting and organizing much information before drafting it.
AI agents can help you with each step along the way, freeing you up to focus on the creative aspects of writing. In this blog, we'll unpack the different types of AI agents and how they can help you write more efficiently to achieve your goals faster.
Otio's AI research and writing partner is a valuable tool to help you achieve your objectives, such as writing and researching efficiently using AI tools. Our AI agent can help you collect and organize research, outline your project, and even draft sections of your paper to help you get started.
Table Of Contents
Importance of AI Agents
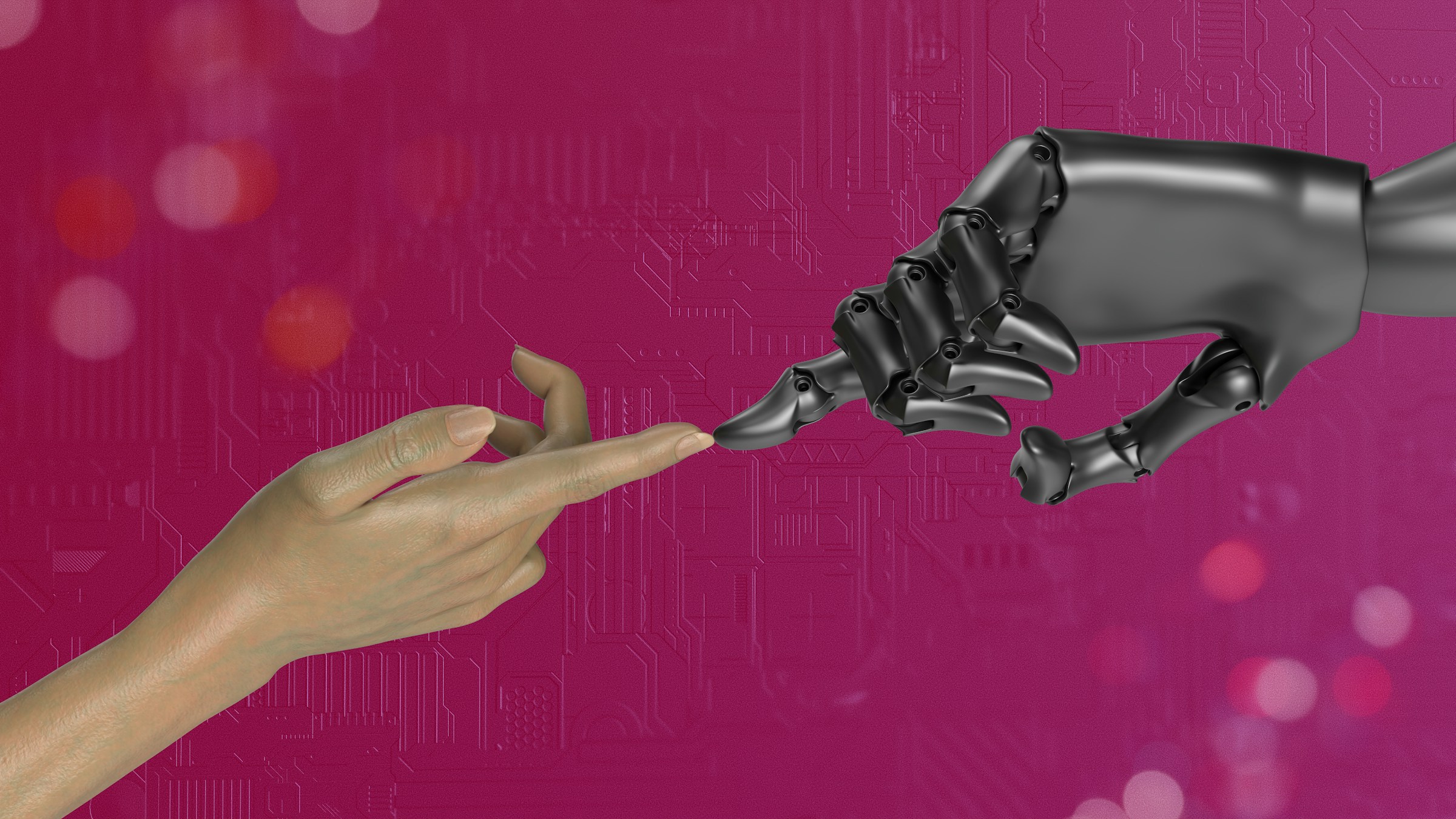
1. AI Agents Improve Operational Efficiency
AI agents significantly enhance operational efficiency. They can automate tedious tasks and optimize resource utilization, fundamentally transforming workflow management. Here’s how they can improve business operations:
Handling Increased Workload
AI agents excel at routine tasks and adapting to evolving business demands. For instance, smart home systems now use AI agents to manage energy consumption and security, replacing outdated timers and alarms for an integrated multi-agent approach. This is just one example: businesses everywhere adopt AI agents for simple and complex tasks.
What Makes AI Agents So Effective?
They automatically manage tasks.
They monitor and adjust operations in real time.
They smoothly integrate with existing systems.
They identify and correct issues before they escalate.
Maintaining Consistent Quality
While humans grow tired as they work, AI agents do not. They can handle much higher workloads than their human counterparts without a drop in performance. Consider hiring a customer service team. They can work efficiently across one time zone. However, as their hours increase, their productivity drops, and so does the quality of their work. AI agents, on the other hand, can maintain their performance, whether it’s 3 AM or 3 PM.
2. They Help Boost Employee Productivity
AI agents improve employee productivity by managing time-consuming, routine tasks. According to research, businesses that implement AI see productivity jump by 40% compared to those that don’t. Newer team members see the most significant boost, with AI pushing their performance up by 43%.
Automating Repetitive Tasks
Every business has monotonous tasks, such as generating invoices, maintaining documents, and replying to emails, that consume a lot of employee time and productivity. AI agents can automate these tedious tasks, freeing up valuable time and resources for more strategic processes.
Pro Tip
Encourage your team to identify their most time-consuming, repetitive tasks. These are prime candidates for AI agent automation and can lead to significant productivity gains.
Reducing Human Error
Human error is inevitable, no matter how careful or well-versed someone is in a process. Even a single mistake, such as billing a customer or handling a high-ticket client, can lead to losses that amount to thousands of dollars. AI agents don’t make these errors as they can’t get tired, bored, or confused in the same process. They can continue doing the same task repeatedly and stay accurate, which helps employees as they don’t have to worry about correcting inaccuracies.
Note
Some AI models can hallucinate, i.e., make up their information, leading to errors. You can avoid these errors using specific prompt engineering techniques and advanced AI tools.
Enabling Focus on Strategic Work
Want to know the best part about AI agents? They free up people to tackle the work that matters. Take customer service teams — while AI handles the routine stuff, agents can focus on solving complex customer issues. AI agents are like productivity partners. They don’t just take over tedious tasks. They help people work smarter and let employees focus on work that needs human creativity and strategic thinking while agents handle mundane tasks. The impact on skill development is another benefit of AI agents. New employees using AI tools can quickly come up to speed with employees having more experience.
3. They Ensure a Better Customer Experience
Not just operational efficiency, the benefit of AI agents is also evident in how they help improve your business’s customer experience. From 24/7 support in multiple languages to personalized interactions, AI agents can transform how you deal with your customers. Did you know 90% of customers expect an immediate response when they reach out with a support query? Anywhere above 10 minutes to respond, and you’re not providing a satisfactory customer experience. If you’re struggling to provide timely customer service, implementing AI agents can be helpful. One of the benefits of AI agents is that they work round-the-clock and are available across time zones. This helps boost customer experience to a great extent, especially with customers who refuse to settle for slower responses.
Personalized Service
Another critical factor in providing excellent customer experiences is to personalize the service. More customers value personalized services; AI agents can help you deliver that. Nearly half of the customers are comfortable with AI agents if it means faster responses, quicker resolutions, and more efficient customer service. While maintaining the human touch is crucial, using AI customer service agents in your business can take a long time to deliver the service experience your customers want.
AI agents analyze customer data, such as purchase history and browsing behavior, to provide personalized experiences. Through machine learning algorithms, intelligent agents can identify patterns in customer behavior and understand their preferences. Then, with the adaptive learning system, AI agents can make recommendations and offer personalized responses to individual customers.
Efficient Query Resolution Through Custom Data
One of the most crucial benefits of AI agents is that you can train them on custom data. Since they can access and train your business’s knowledge base, these agents can quickly fetch and analyze the required data to offer accurate on-brand responses to common customer queries. The virtual financial assistant Erica is one of the best examples of AI agents' effectiveness. Introduced by Bank of America, Erica has solved customer queries over the past six years, providing personalized solutions to over 2 billion users.
4. They Help Save Costs
Many AI agents are cost-effective to implement. AI agents cost much less than hiring multiple human employees and deliver better productivity. After bringing AI solutions, 39% of organizations see significant cost reductions in various business functions. And it’s not just due to higher productivity. Here are multiple ways in which AI agents benefit businesses in saving expenses:
Daily operations cost less with automation.
Customer service runs 24/7 without overtime.
Training new staff gets cheaper and faster.
Fewer mistakes mean lower costs.
Teams and resources work smarter.
From finances and marketing to customer service and human resources, AI agents can contribute to cost savings across departments.
5. They Boost Scalability and Flexibility
AI agents can process large volumes of data and handle any workload if you configure them right. That means they are scalable, flexible, and adapt to different “situations” or “processes.” This benefit of AI agents has helped several businesses expand while keeping expenses in check. Say you hire multiple human employees to do the same tasks. Apart from their salaries, you’ll also need to spend time and resources to train them, incur costs to provide them with working space and equipment, and keep an eye on their performance—all this with the additional risk of employee turnover. But scaling with AI agents comes without these extra costs.
They produce consistent, high-quality output regardless of how much workload is assigned. You can also quickly scale them. A brief discussion with your AI agent provider or a switch to a higher plan is all you need to get more resources at hand. You’ll also get better options for scaling the agents at much lesser costs than scaling manually. Companies like Microsoft are already building and deploying agents to help businesses scale. Microsoft is launching 10 new AI agents for sales, supply, finance, and customer service in Dynamics 365. These agents will enable companies to move from traditional scaling methods to a more AI-first approach.
6. They Bring Data-Driven Insights
AI agents can collect and analyze big data in minutes. That makes these intelligent assistants indispensable for business owners making data-driven decisions. Apart from that, you can use AI agents to create reports on market analysis and customer behavior analysis.
Real-time Analytics and Reporting
Using AI and automation for data analysis and reporting isn’t new. Companies have been doing it for a long time, moving from the usual Excel sheets to a more sophisticated and accurate approach. But AI agents take it a step further. They can analyze large datasets quickly and offer real-time insights to help businesses scale operations effectively. With an intelligent AI agent, your marketing or sales team can identify trends and make strategic decisions within minutes — something that’ll save you time and put you ahead of competitors.
Pattern Recognition and Trends
Recognizing patterns and trends is integral to making key business decisions, especially regarding product launches, sales, and content creation. And when there are massive data sets, observing these trends can be tricky, even with straightforward automation. AI agents can identify patterns in large datasets, even those too complex for humans to notice.
By analyzing historical data and real-time inputs, they can uncover trends that help your business stay ahead of the curve. Take an e-commerce business, for instance. AI e-commerce agents can analyze purchasing behaviors to predict customer preferences, seasonal demand, or potential churn risks. In finance, they can detect spending patterns to spot fraudulent transactions before they escalate. By recognizing these patterns and trends, you can make smarter, data-driven decisions — optimizing inventory, personalizing marketing campaigns, or identifying future growth opportunities.
7. They Give a Competitive Advantage
Want to stay one step ahead of your competitors? AI agents ensure you’re always up-to-date with their innovation and marketing capabilities.
Innovation Capabilities
AI agents aren’t just about speed — they’re changing how companies innovate. Research shows that AI adoption significantly enhances organizational capabilities regarding employee innovation, creativity, and experimentation. Many tech companies have already adopted AI agents to potential their innovation strategies with:
Quick testing of new ideas
Smarter problem-solving
Better team collaboration
Faster R&D cycles
The best part? Innovation isn’t just for big companies. Due to the cost-effectiveness of AI agents, many smaller companies are already using them for automation, analytics, and customer service and are seeing great results.
Marketing Capabilities
Marketing is also getting more manageable, a key benefit of AI agents. Whether writing ad copy or creating full-fledged go-to-market (GTM) strategies, AI agents can speed up the marketing process and fuel creativity.
Types of AI Agents
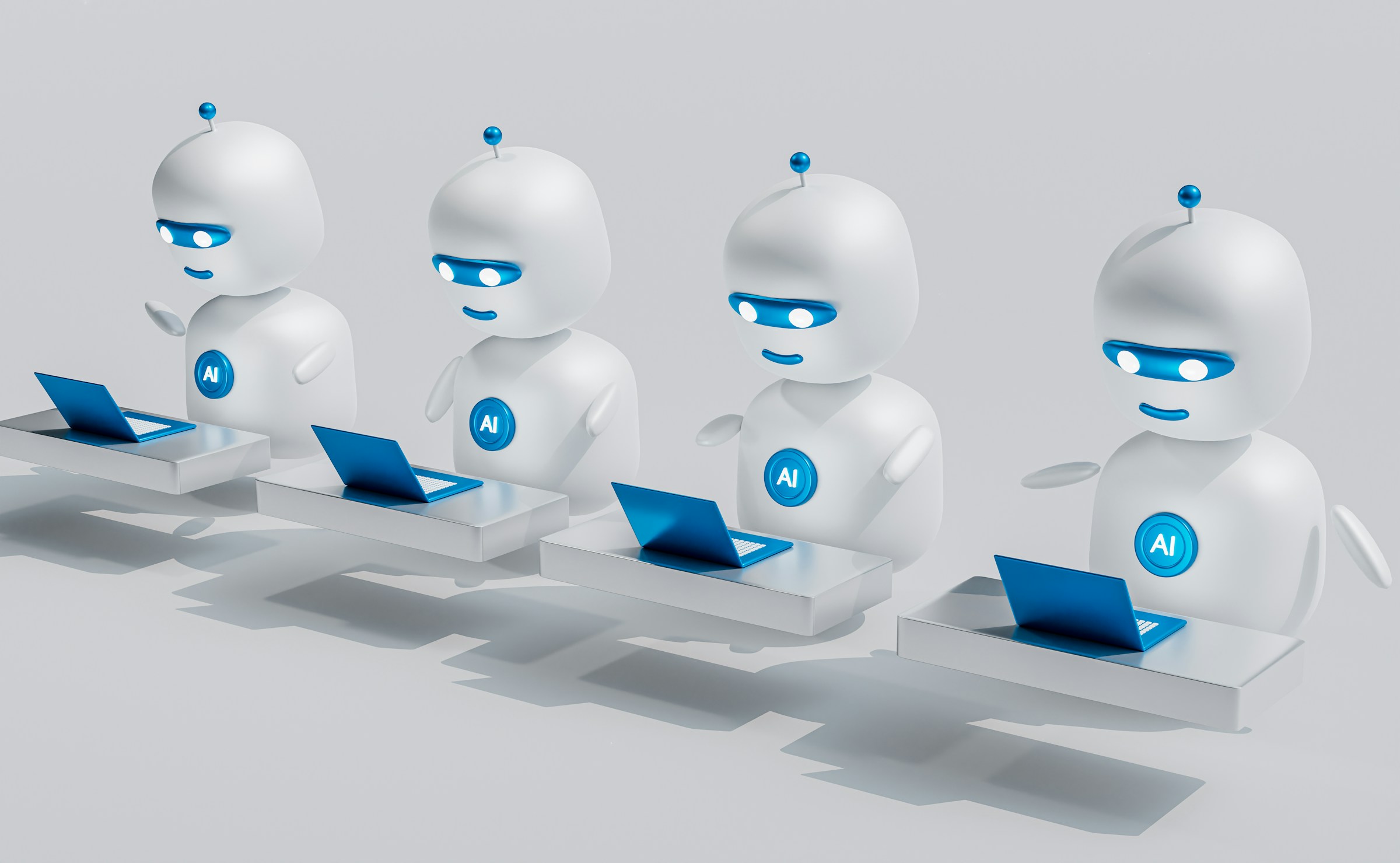
1. Simple Reflex Agents: The Fundamental Building Blocks of AI
Simple reflex agents represent one of the most elementary forms of artificial intelligence. These agents make decisions based solely on their current sensory input, responding immediately to environmental stimuli without needing memory or learning processes. Their behavior is governed by predefined condition-action rules, which specify how to react to particular inputs. Though they are limited in complexity, this straightforward approach makes them highly efficient and easy to implement, especially in environments where the range of possible actions is limited.
Key components
Sensors: Much like human senses, these gather environmental information. Sensors are essential input devices for a simple reflex agent that detects specific environmental conditions like temperature, light, or motion.
Condition-action rules: These predefined rules determine how the agent responds to specific inputs. The logic is direct—if the agent detects a particular condition, it immediately performs a corresponding action.
Actuators: These execute the decisions made by the agent, translating them into physical or digital responses that alter the environment in some way, such as activating a heating system or turning on lights.
Use cases
Simple reflex agents are ideal for transparent, predictable environments with limited variables.
Industrial safety sensors that immediately shut down machinery when detecting an obstruction in the work area.
Automated sprinkler systems that activate based on smoke detection.
Email auto-responders that send predefined messages based on specific keywords or sender addresses.
2. Model-Based Reflex Agents: The Next Level of AI
Model-based reflex agents improve on the simple reflex design by maintaining an internal model of the world, allowing them to operate in more complex, less predictable environments. Unlike simple reflex agents, which react solely based on current sensory input, model-based agents maintain an internal representation, or model, of the world. This model tracks how the environment evolves, allowing the agent to infer unobserved aspects of the current state. While these agents don’t actually “remember” past states like more advanced agents do, they use their world model to make better decisions about the current state.
Key components
State tracker: Maintains information about the current state of the environment based on the world model and sensor history.
World model: Contains two key types of knowledge: how the environment evolves independent of the agent and how the agent’s actions affect the environment.
Reasoning component: Uses the world model and current state to determine appropriate actions based on condition-action rules.
Use cases
These agents are suitable for environments where the current state isn’t fully observable from sensor data alone.
Smart home security systems: Using models of standard household activity patterns to distinguish between routine events and potential security threats.
Quality control systems: Monitoring manufacturing processes by maintaining a model of normal operations to detect deviations.
Network monitoring tools: Tracking network state and traffic patterns to identify potential issues or anomalies.
3. Goal-Based Agents: AI with a Purpose
Goal-based agents are designed to pursue specific objectives by considering the future consequences of their actions. Unlike reflex agents that act based on rules or world models, goal-based agents plan sequences of actions to achieve desired outcomes. They use search and planning algorithms to find action sequences that lead to their goals.
Key components
Goal state: A clear description of the agent's goals.
Planning mechanism: The ability to search through possible sequences of actions that could lead to the goal.
State evaluation: Methods to assess whether potential future states move closer to or further from the goal.
Action selection: The process of choosing actions based on their predicted contribution toward reaching the goal.
World model: Understanding how actions change the environment, used for planning.
Use cases
Goal-based agents are suited for tasks with clear objectives and predictable action outcomes.
Industrial robots: Following specific sequences to assemble products.
Automated warehouse systems: Planning optimal paths to retrieve items.
Smart heating systems: Planning temperature adjustments to reach desired comfort levels efficiently.
Inventory management systems: Planning reorder schedules to maintain target stock levels.
Task scheduling systems: Organizing sequences of operations to meet completion deadlines.
4. Learning Agents: AI That Gets Smarter Over Time
A learning agent is an artificial intelligence system capable of improving its behavior over time by interacting with its environment and learning from its experiences. These agents modify their behavior based on feedback and experience, using various learning mechanisms to optimize performance. Unlike more straightforward agent types, they can discover how to achieve their goals through experience rather than purely relying on pre-programmed knowledge.
Key components:
Performance element: The component that selects external actions, similar to the decision-making modules in more straightforward agents.
Critic: Provides feedback on the agent’s performance by evaluating outcomes against standards, often using a reward or performance metric.
Learning element: Uses critic’s feedback to improve the performance element, determining how to modify behavior to do better in the future.
Problem generator: Suggests exploratory actions that lead to new experiences and better future decisions.
Use cases
Learning agents are suited for environments where optimal behavior isn’t known in advance and must be learned through experience.
Industrial process control: Learning optimal settings for manufacturing processes through trial and error.
Energy management systems: Learning patterns of usage to optimize resource consumption.
Customer service chatbots: Improving response accuracy based on interaction outcomes.
Quality control systems: Learning to identify defects more accurately over time.
5. Utility-Based Agents: Striking a Balance
A utility-based agent makes decisions by evaluating the potential outcomes of its actions and choosing the one that maximizes overall utility. Unlike goal-based agents that aim for specific states, utility-based agents can handle tradeoffs between competing goals by assigning numerical values to different outcomes.
Key components
Utility function: A mathematical function that maps states to numerical values, representing the desirability of each state.
State evaluation: Methods to assess current and potential future states regarding their utility.
Decision mechanism: Processes for selecting actions that are expected to maximize utility.
Environment model: Understanding of how actions affect the environment and resulting utilities.
Use cases
Utility-based agents are suited for scenarios requiring a balance between multiple competing objectives.
Resource allocation systems: Balancing machine usage, energy consumption, and production goals.
Smart building management: Optimizing between comfort, energy efficiency, and maintenance costs.
Scheduling systems: Balancing task priorities, deadlines, and resource constraints.
6. Hierarchical Agents: AI with a Chain of Command
Hierarchical agents are structured in a tiered system, where higher-level agents manage and direct the actions of lower-level agents. This architecture breaks down complex tasks into manageable subtasks, allowing for more organized control and decision-making.
Key components
Task decomposition: Break down complex tasks into simpler subtasks that lower-level agents can manage.
Command hierarchy: Defines how control and information flow between different levels of agents.
Coordination mechanisms: Ensures different levels of agents work together coherently.
Goal delegation: Translates high-level objectives into specific tasks for lower-level agents.
Use cases
Hierarchical agents are best suited for systems with clear task hierarchies and well-defined subtasks.
Manufacturing control systems: Coordinating different stages of production processes.
Building automation: Managing basic systems like HVAC and lighting through layered control.
Robotic task planning: Breaking down simple robotic tasks into basic movements and actions.
7. Multi-Agent Systems: A Whole Team Working Toward a Goal
A multi-agent system involves multiple autonomous agents interacting within a shared environment, working independently or cooperatively to achieve individual or collective goals. While often confused with more advanced AI systems, traditional MAS focuses on simple agents interacting through basic protocols and rules.
Types of multi-agent systems
Cooperative systems: Agents share information and resources to achieve common goals—for example, multiple robots work together on basic assembly tasks.
Competitive systems: Agents compete for resources following defined rules, like multiple bidding agents in a simple auction system.
Mixed systems: Combines both cooperative and competitive behaviors, such as agents sharing some information while competing for limited resources.
Key components
Communication protocols: Define how agents exchange information.
Interaction rules: Specify how agents can interact and what actions are permitted.
Resource management: Methods for handling shared resources between agents.
Coordination mechanisms: Systems for organizing agent activities and preventing conflicts.
Use cases
MAS best suits scenarios with clear interaction rules and relatively simple agent behaviors.
Warehouse management: Multiple robots coordinate to move and sort items.
Basic manufacturing: Coordinating simple assembly tasks between multiple machines.
Resource allocation: Managing shared resources like processing time or storage space.
Related Reading
• Workflow Examples
• Marketing Automation Workflow
• AI Workflow Automation
• AI That Can Search the Web
• Marketing Automation Workflow Examples
11 Real-Life AI Agent Examples
1. Otio: The AI Research Assistant for Content Overload

Knowledge workers, researchers, and students are overwhelmed by content overload and left to deal with it using fragmented, complex, and manual tooling. Otio solves this problem by providing researchers with one AI-native workspace. It helps them:
Collect many data sources, from bookmarks, tweets, and extensive books to YouTube videos.
Extract key takeaways with detailed AI-generated notes and source-grounded Q&A chat.
Create draft outputs using the sources you’ve collected. Otio helps you to go from the reading list to the first draft faster.
Along with this, Otio also enables you to write research papers/essays faster. Here are our top features that researchers love: AI-generated notes on all bookmarks (Youtube videos, PDFs, articles, etc.), Otio enables you to chat with individual links or entire knowledge bases, just like you chat with ChatGPT, as well as AI-assisted writing.
Let Otio be your AI research and writing partner -- try Otio for free today!
2. ChatGPT by OpenAI: The AI for Writing & Customer Support
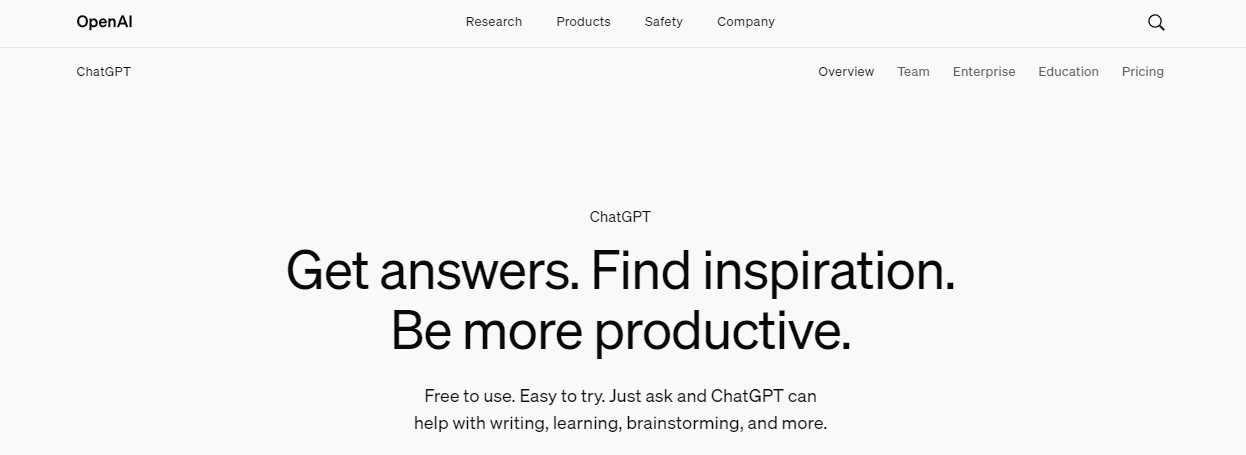
ChatGPT is an AI chatbot that generates human-like responses to user prompts. Businesses use ChatGPT to automate customer support, conversations, content writing, and brainstorming ideas. For example, companies like Shopify integrate ChatGPT for automated replies to customer inquiries, saving time and improving customer satisfaction.
3. Replika: An AI Companion
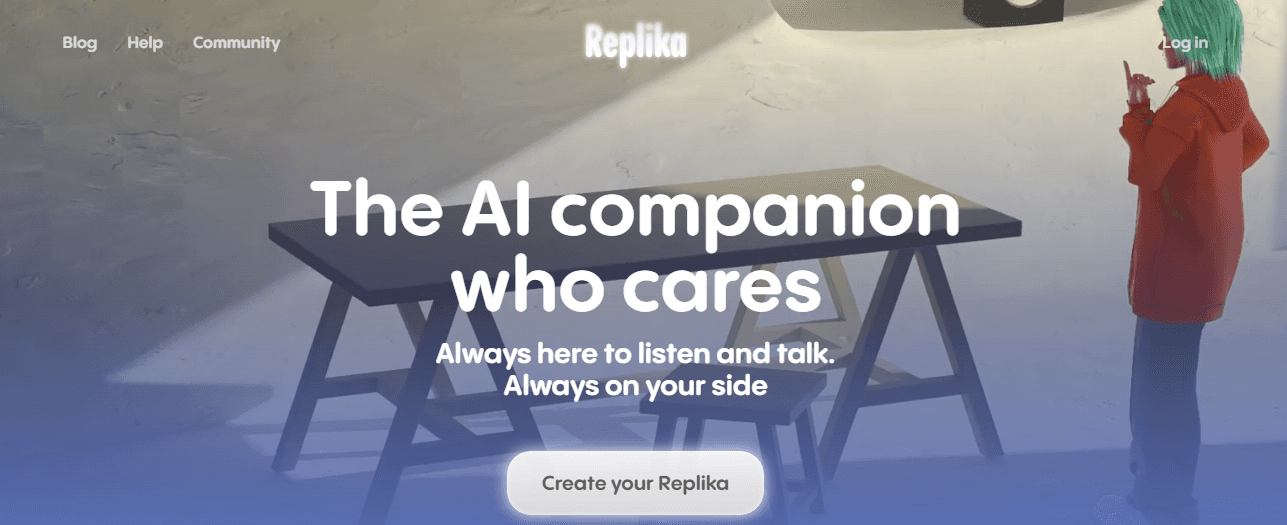
Replika is an AI chatbot that offers companionship and mental health support. Users interact with Replika to build relationships, vent, or seek advice, helping reduce loneliness.
4. Tesla Autopilot: Self-Driving Cars
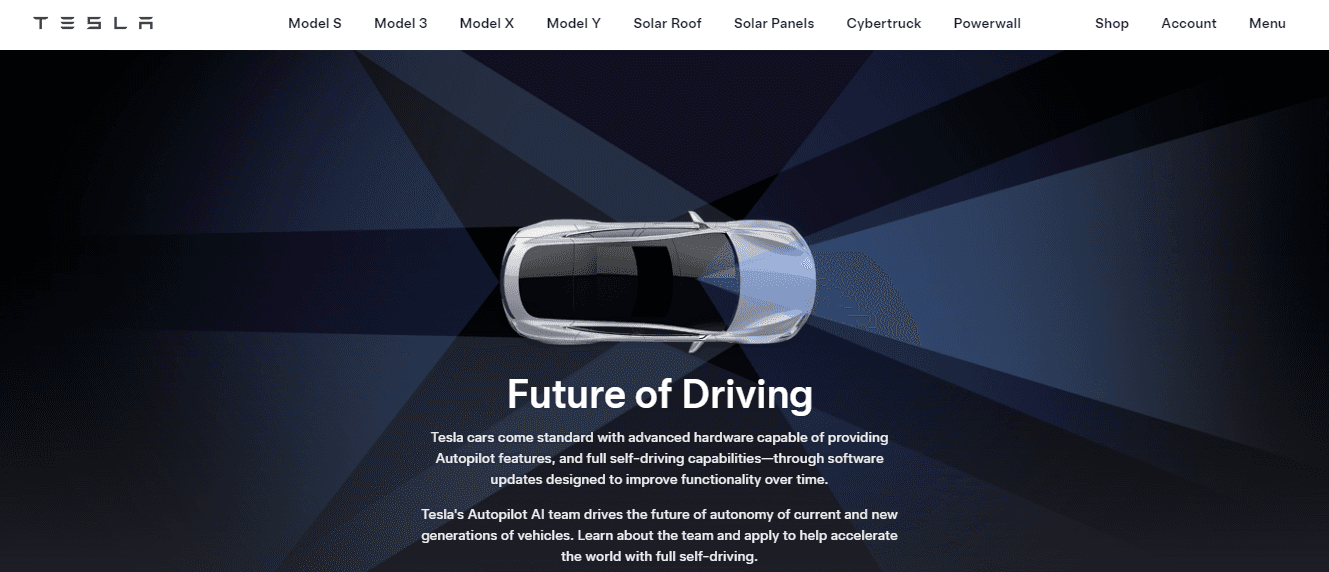
Tesla Autopilot is an advanced driver-assistance system that uses AI agents to process road conditions, detect objects, and make driving decisions. For instance, Tesla's AI agent analyzes real-time traffic data to enable hands-free highway driving.
5. Amelia by IPsoft: Enterprise AI for Customer Service
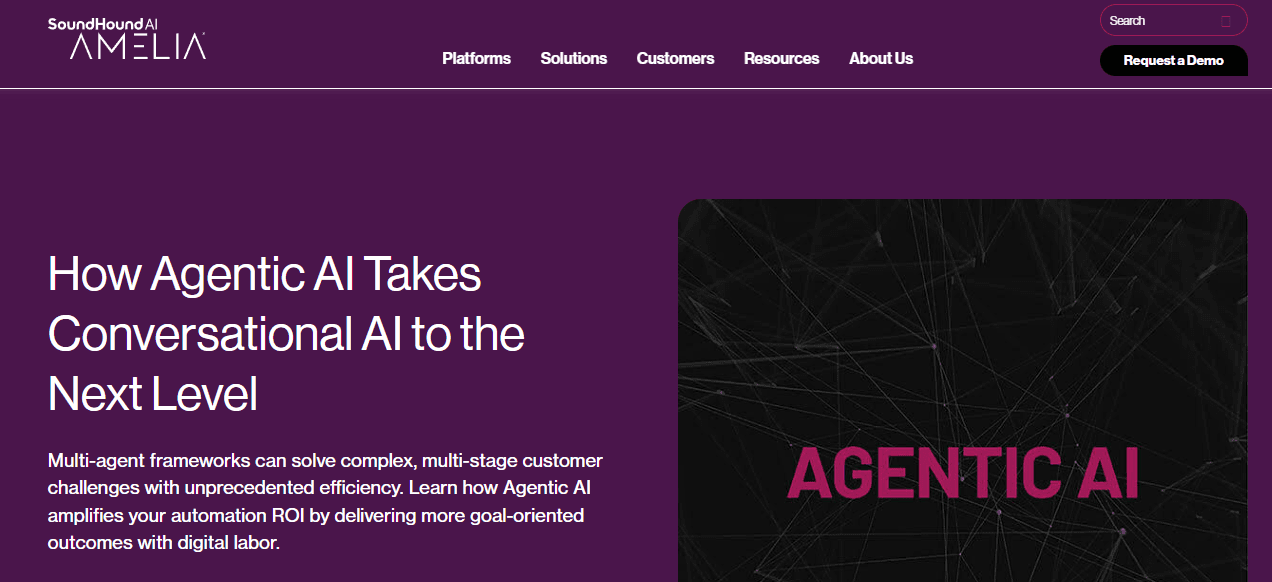
Amelia is a conversational AI that automates customer service and IT operations. For example, Amelia assists banking customers by processing transactions and troubleshooting issues without human intervention.
6. Google Assistant: The Personal AI Assistant
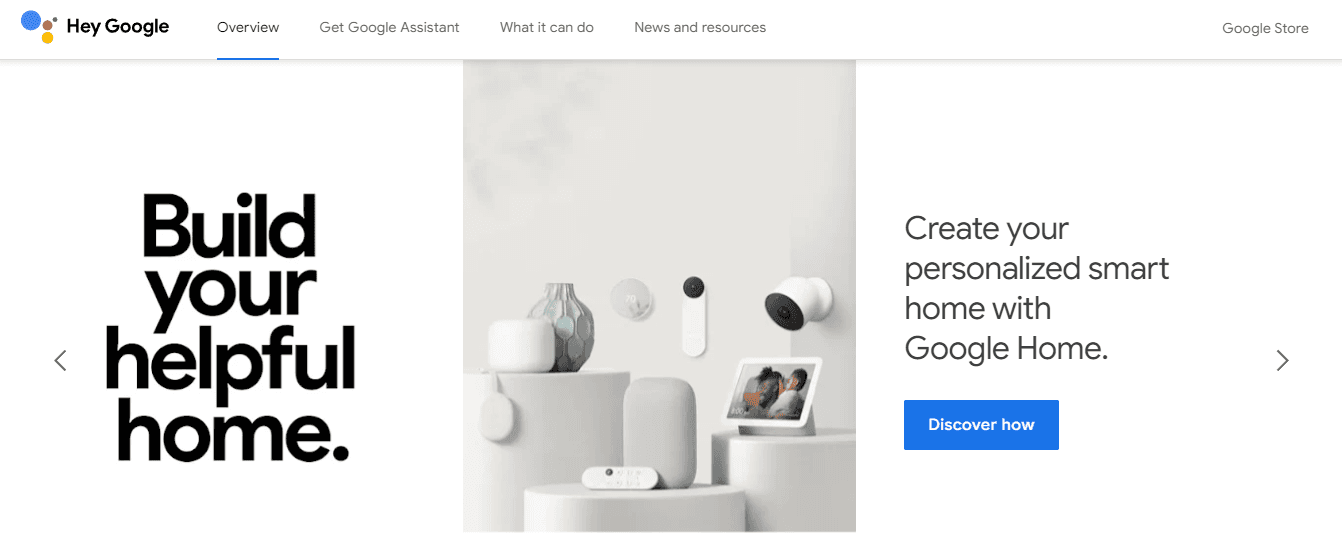
Google Assistant is an AI that helps users manage their day-to-day tasks and smart home devices. For example, Google Assistant integrates with Nest devices for automated lighting, thermostat control, and reminders.
7. IBM Watson Assistant: AI for Healthcare & Banking
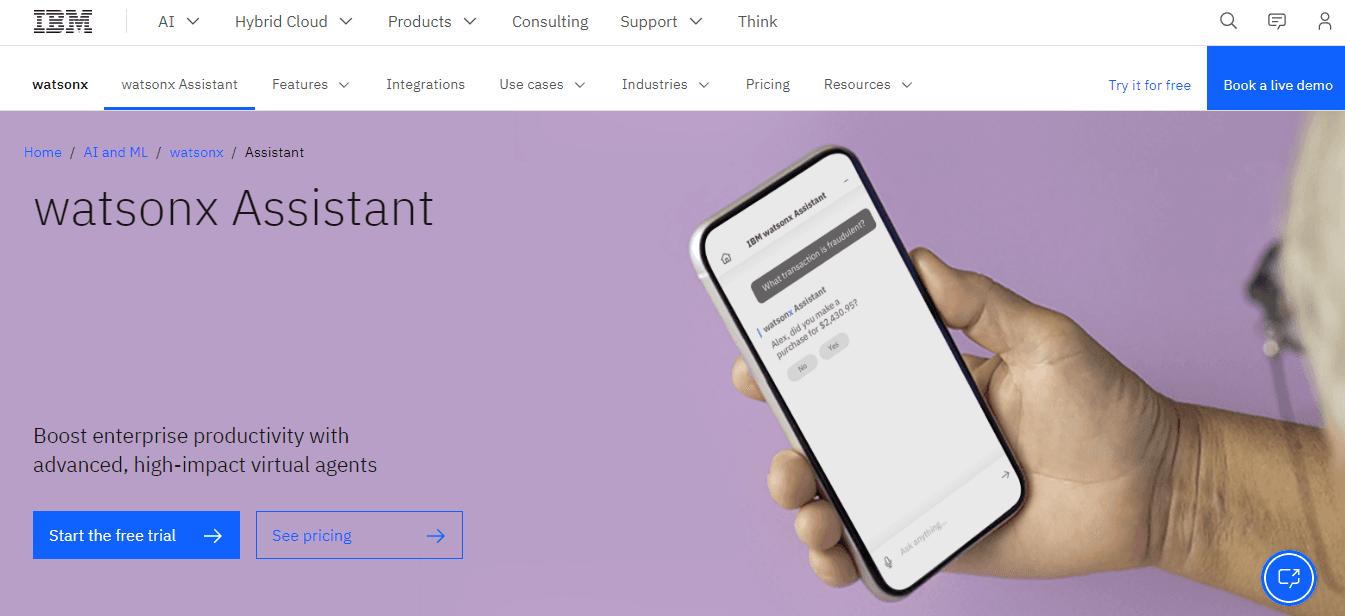
IBM Watson Assistant is an AI agent that provides personalized healthcare and banking inquiry recommendations. For example, Watson could use Cleveland Clinic chatbots to assist patients with appointments and post-care instructions.
8. Amazon Alexa: Smart Home Ecosystems
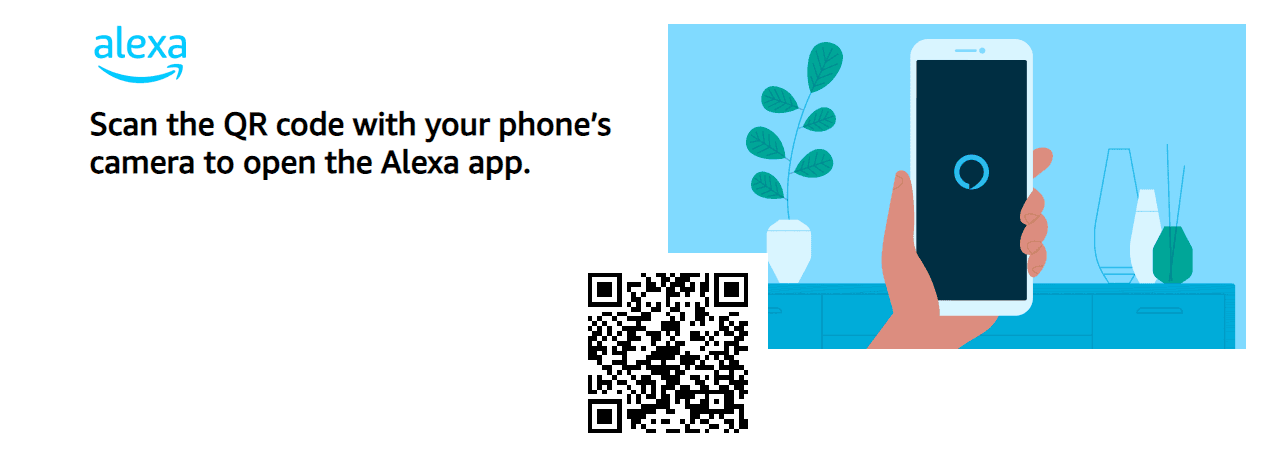
Amazon Alexa is an AI voice assistant that controls smart devices, answers questions, and streams entertainment. For instance, Alexa manages entire home ecosystems, from playing music to reordering groceries through voice commands.
9. UiPath Robots: RPA for Business Automation
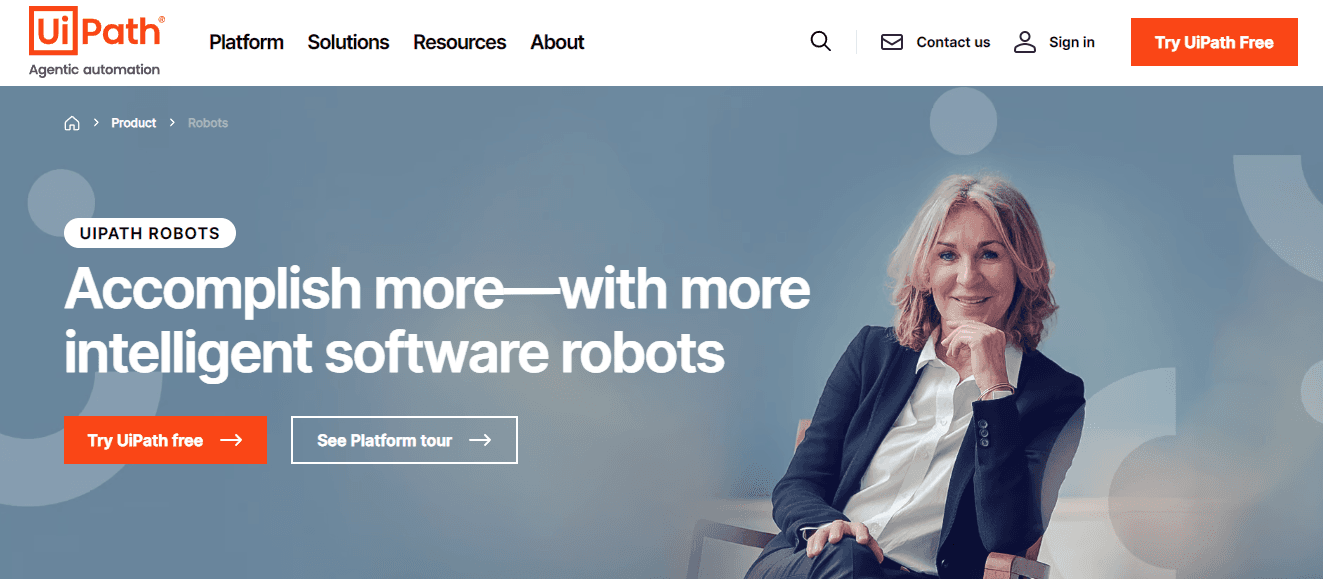
UiPath Robots are AI-powered robotic process automation (RPA) agents that handle repetitive finance, HR, and logistics tasks. For example, UiPath bots process invoices and payroll at scale, reducing manual workload.
10. OpenAI Codex: AI for Programming Assistance
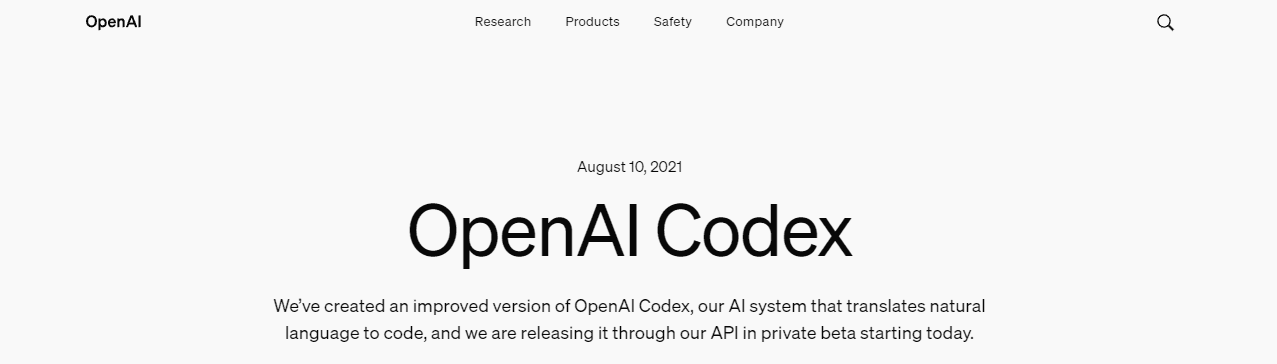
OpenAI Codex is an AI agent that generates code based on natural language prompts. For example, GitHub Copilot uses Codex to suggest code snippets and debug software for developers.
11. DeepMind AlphaFold: AI for Scientific Discovery
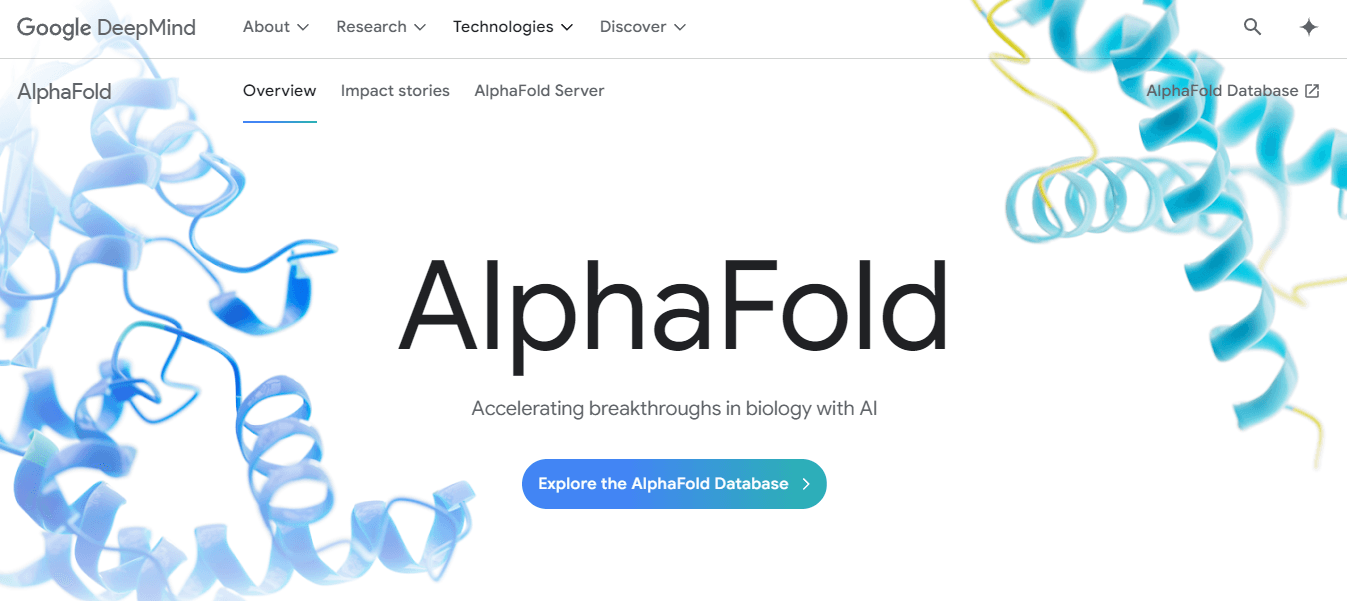
DeepMind AlphaFold is an AI that accurately predicts protein structures, transforming biology and medicine. For example, AlphaFold aids in drug discovery by providing insights into complex protein folding problems.
Related Reading
• AI Business Automation
• How to Automate Tasks
• How to Build Workflows
• Workflow Automation Tools
• Document Workflow Automation
• AI Productivity Tools
• Workflow Apps
• How to Use AI to Automate Tasks
• AI Workflows
• CRM Workflow Automation
• AI Automation Tools
How to Leverage AI Agents
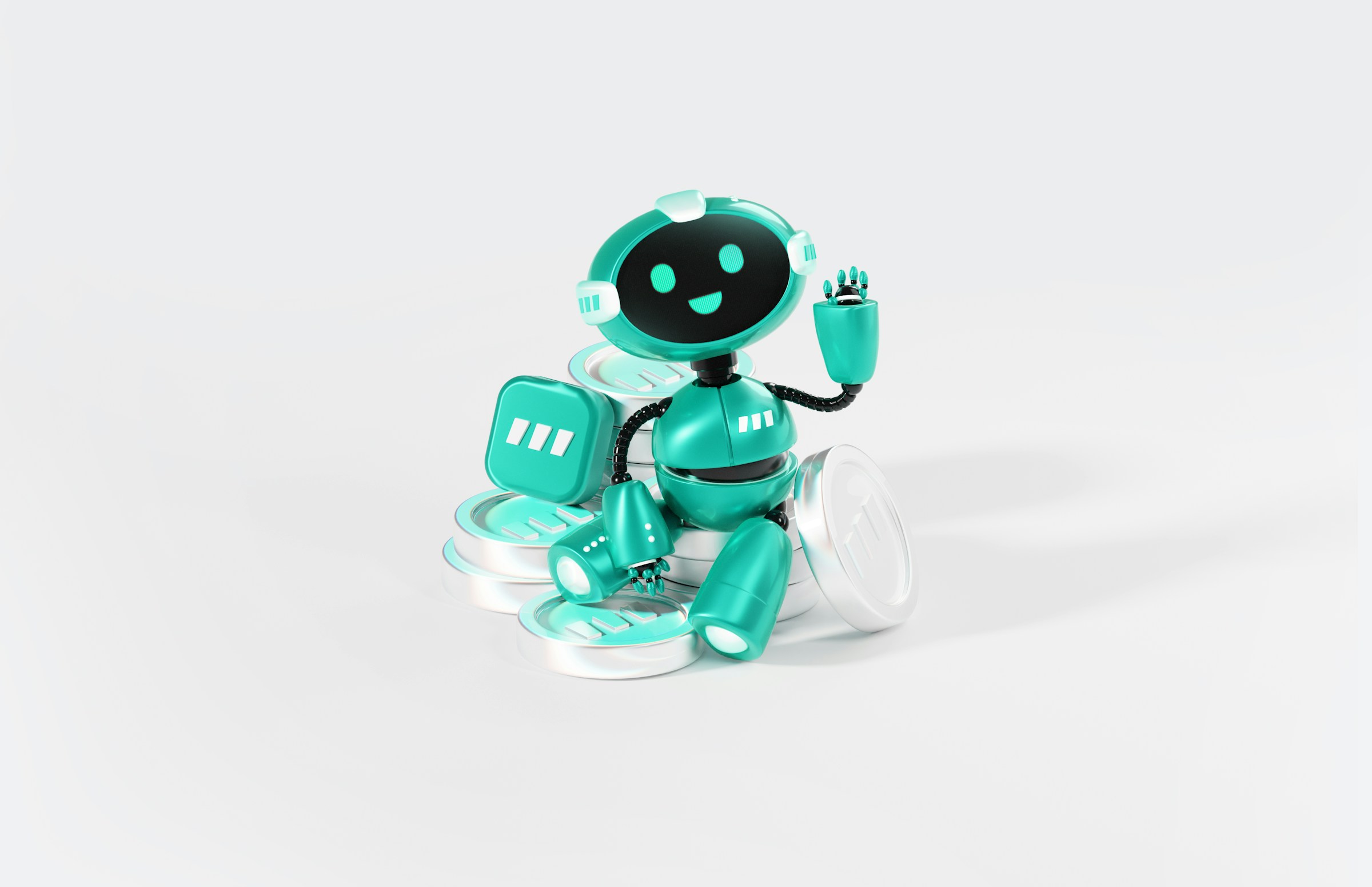
Optimize Resource Management with AI Agents
AI agents can effectively adjust resources as demands change. They analyze traffic patterns, application performance, and user behavior. For instance, when a new product launches, AI agents ensure that cloud resources scale to accommodate the visitor surge. Once traffic returns to normal, resources can go back to baseline levels. This use of AI helps organizations better manage changing workloads. It improves user experience and helps keep costs down.
Bolster Security with AI Agents
AI agents can also enhance security. They examine activity logs and monitor system behavior in real-time. This way, they can detect anomalies and flag potential threats before they escalate. This proactive approach to identifying threats helps reduce risks and protects sensitive data, even in dynamic cloud environments.
Improve Development with AI Agents
The development phase usually includes repetitive tasks like writing test cases, debugging code, and preparing for deployments. These manual processes slow productivity, introduce errors, and raise costs. AI agents help alleviate repetitive work by automating it and providing valuable insights. For example, testing teams can use generative AI agents to automate test case creation.
This enables comprehensive coverage of new features without extensive manual work. Additionally, these agents can recommend configuration changes and optimizations by analyzing historical data, helping improve the overall quality of applications. Their ability to provide real-time feedback allows developers to identify problems quickly without waiting for scheduled reviews. This rapid response speeds up development and helps ensure the final product is robust and reliable.
Enable Intelligent Decision-Making in DevOps
One major strength of AI agents is their ability to make wise decisions autonomously. They utilize collected data along with their internal model of the world to evaluate options and determine the best course of action.
Let Otio be your AI research and writing partner -- try Otio for free today!
Knowledge workers, researchers, and students are overwhelmed by content overload and left to deal with it using fragmented, complex, and manual tooling. Otio solves this problem by providing researchers with one AI-native workspace. It helps them:
Collect many data sources, from bookmarks, tweets, and extensive books to YouTube videos.
Extract key takeaways with detailed AI-generated notes and source-grounded Q&A chat.
Create draft outputs using the sources you’ve collected. Otio helps you to go from the reading list to the first draft faster.
Along with this, Otio also enables you to write research papers/essays faster. Here are our top features that researchers love: AI-generated notes on all bookmarks (Youtube videos, PDFs, articles, etc.), Otio enables you to chat with individual links or entire knowledge bases, just like you chat with ChatGPT, as well as AI-assisted writing.
Supercharge Your Researching Ability With Otio — Try Otio for Free Today
Knowledge workers, researchers, and students are overwhelmed by content overload and left to deal with it using fragmented, complex, and manual tooling. Otio solves this problem by providing researchers with one AI-native workspace. It helps them:
Collect many data sources, from bookmarks, tweets, and extensive books to YouTube videos.
Extract key takeaways with detailed AI-generated notes and source-grounded Q&A chat.
Create draft outputs using the sources you’ve collected. Otio helps you to go from the reading list to the first draft faster.
Along with this, Otio also enables you to write research papers/essays faster. Here are our top features that researchers love: AI-generated notes on all bookmarks (Youtube videos, PDFs, articles, etc.), Otio enables you to chat with individual links or entire knowledge bases, just like you chat with ChatGPT, as well as AI-assisted writing.
Let Otio be your AI research and writing partner -- try Otio for free today!
Related Reading
• HR Workflow Automation
• Best AI Agents
• Workflow Automation for Small Business
• Marketing Workflow Automation
• Low Code Workflow Automation
• Sales Workflow Automation
• AI Workflow Tools
• AI Financial Statement Analysis
• AI Tools for Finance