AI Workflow Management
40 AI Agent Examples for Different Industries
Explore 40+ AI agent examples across industries, showcasing real-world applications and innovations in automation and problem-solving.
Jan 17, 2025
Consider approaching a school assignment or research paper with a fresh perspective, free from anxiety and self-doubt. What if a virtual assistant could help you brainstorm, plan, write, and even edit your paper, guiding you as you go and taking care of the boring stuff? This is one of the many benefits of AI Workflow management. In this guide, we’ll introduce you to AI agents and explore examples of how they can help students, particularly with writing and research tasks.
One AI agent, Otio's AI research and writing partner, can help you reach your academic goals by making the research and writing processes faster, easier, and more efficient. The tool will help you organize and even suggest improving your writing.
Table Of Contents
How Do AI Agents Work

An artificial intelligence agent is a system or program that autonomously performs tasks on a user's or another system's behalf. AI agents design their workflows, utilizing available tools to accomplish tasks. These agents encompass various functionalities beyond natural language processing, including decision-making, problem-solving, interacting with external environments, ts and executing actions. AI agents can be deployed in various applications to solve complex enterprise-level tasks, from software design and IT automation to conversational assistants. They use advanced natural language processing techniques of large language models to comprehend and respond to user inputs step-by-step and determine when to call on external tools.
The Role of Large Language Models in AI Agent Technology
At the core of AI, agents are large language models. For this reason, AI agents are often referred to as LLM agents. Traditional LLMs, such as IBM® Granite™ models, produce their responses based on the data used to train them and are bounded by knowledge and reasoning limitations. In contrast, agentic technology uses tools called on the backend to obtain up-to-date information and create subtasks autonomously to achieve complex goals.
How AI Agents Work: Three-Step Process
The approach that AI agents take in achieving goals set by users is comprised of three stages:
Goal initialization and planning
Reasoning using available tools
Learning and reflection
1. Goal Initialization and Planning
Although AI agents are autonomous in decision-making, they require goals and environments humans define. There are three main influences on autonomous agent behavior:
The team of developers designs and trains the agentic AI system.
The team that deploys the agent and provides the user access to it.
The user provides the AI agent with specific goals and establishes available tools.
Given the user’s goals and available tools, the AI agent performs task decomposition to improve performance. Essentially, the agent creates a plan of specific tasks and subtasks to accomplish the complex goal. For simple tasks, planning is not a necessary step. Instead, an agent can iteratively reflect on and improve responses without planning its next steps.
2. Reasoning Using Available Tools
AI agents base their actions on the information they perceive. Often, AI agents lack the knowledge base needed to tackle all subtasks within a complex goal. To remedy this, AI agents use their available tools. These tools can include external data sets, web searches, APIs, and even other agents. After the missing information is retrieved from these tools, the agent can update its knowledge base. This means that each step of the way, the agent reassesses its action plan and self-corrects. To help illustrate this process, imagine a user planning their vacation.
The user tasks an AI agent with predicting which week in the next year would likely have the best weather for their surfing trip in Greece. Since the LLM model at the agent's core does not specialize in weather patterns, the agent gathers information from an external database of daily weather reports for Greece over the past several years. Despite acquiring this new information, the agent still cannot determine the optimal weather conditions for surfing, so the next subtask has been created. For this subtask, the agent communicates with an external agent that specializes in surfing.
In doing so, the agent learns that high tides and sunny weather with little to no rain provide the best surfing conditions. The agent can now combine the information it has learned from its tools to identify patterns. It can predict which week in Greece will likely have high tides, sunny weather, and a low chance of rain next year. These findings are then presented to the user. This information-sharing between tools allows AI agents to be more general-purpose than traditional AI models.
3. Learning and Reflection
AI agents use feedback mechanisms, such as other AI agents and human-in-the-loop, to improve the accuracy of their responses. Let’s return to our previous surfing example to highlight this. After the agent forms its response to the user, the agent stores the learned information along with the user’s feedback to improve performance and adjust to user preferences for future goals. If other agents were used to reach the goal, their feedback may also be used. Multi-agent feedback can be instrumental in minimizing human users' time providing direction. However, users can also provide input throughout the agent’s actions and internal reasoning to better align the results with the intended goal. Feedback mechanisms improve the AI agent’s reasoning and accuracy, commonly called iterative refinement. AI agents can also store data about solutions to previous obstacles in a knowledge base to avoid repeating the same mistakes.
Benefits of AI Agents for Companies
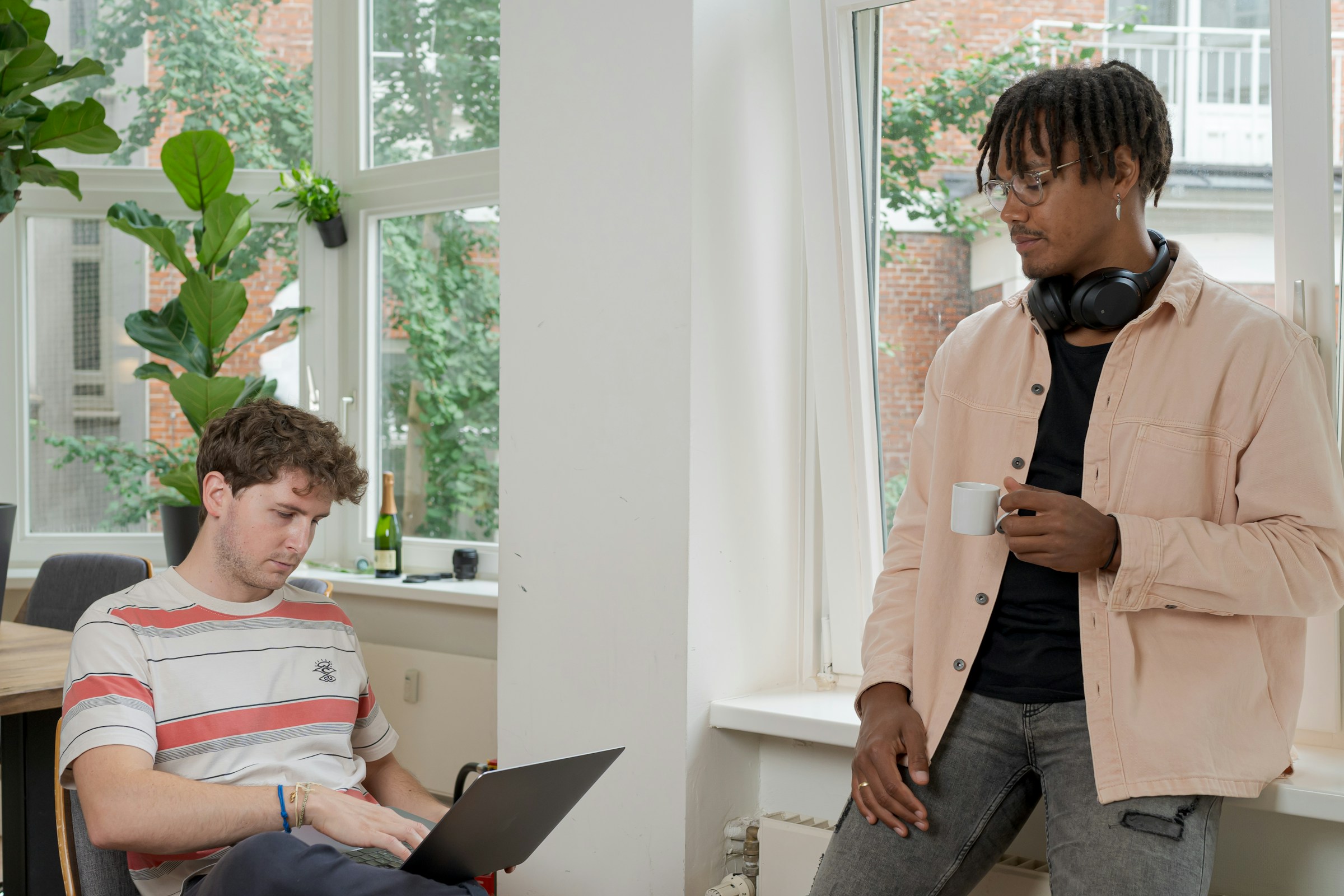
AI Agents Boost Operational Efficiency
AI agents significantly improve operational efficiency. These intelligent agents automate tedious tasks and help organizations manage workflows more effectively. For example, smart home systems have switched from timers and alarms to AI agents that manage energy consumption and security. Businesses can use AI agents for both simple and complex tasks. Their effectiveness comes from:
Automating task management
Monitoring and adjusting operations in real-time
Integrating smoothly with existing systems
Spotting and fixing issues before they escalate
AI agents maintain consistent quality, too. Unlike humans, they don’t get tired. So, operational quality is assured no matter how much or how long AI agents work.
AI Agents Improve Employee Productivity
AI agents boost employee productivity by handling time-consuming, repetitive tasks. One study found businesses that use AI tools see productivity jump by 40% compared to those that don’t. Newer team members see the most significant gains, with AI pushing their performance up by 43%. For instance, every business has monotonous tasks that take up valuable employee time. Whether generating invoices, maintaining documents, or replying to emails, these tasks can destroy productivity. What is the key benefit of AI agents? They help automate those tedious tasks, freeing your valuable time and resources for more useful processes.
AI Agents Ensure a Better Customer Experience
The benefits of AI agents extend well beyond operational efficiency. They also help improve your business’s customer experience. From 24/7 support in multiple languages to personalized interactions, AI agents can transform how you deal with your customers.
Did you know 90% of customers expect an immediate response when they reach out with a support query? Implementing AI agents can be helpful if you can’t provide timely customer service. One of the benefits of AI agents is that they work round-the-clock and are available across time zones. This helps boost customer experience to a great extent, especially with customers who refuse to settle for slower responses.
AI Agents Help Save Costs
Many AI agents are cost-effective to implement. AI agents cost much less than hiring multiple human employees and deliver better productivity. After bringing AI solutions, 39% of organizations see significant cost reductions across various business functions.
And it’s not just due to higher productivity. Here are multiple ways in which AI agents benefit businesses in saving expenses:
Daily operations cost less with automation
Customer service runs 24/7 without overtime
Training new staff gets cheaper and faster
Fewer mistakes mean lower costs
Teams and resources work smarter
AI Agents Boost Scalability and Flexibility
AI agents can process large volumes of data and handle any workload if you configure them right. That means they are scalable, flexible, and adapt to different “situations” or “processes.” This benefit of AI agents has helped several businesses expand while keeping expenses in check. Say you hire multiple human employees to do the same tasks.
Apart from their salaries, you’ll also need to spend time and resources to train them, incur costs to provide them with working space and equipment and keep an eye on their performance, with the additional risk of employee turnover. But scaling with AI agents comes without these extra costs.
They produce consistent, high-quality output regardless of how much workload is assigned. You can also quickly scale them. A brief discussion with your AI agent provider or a switch to a higher plan is all you need to get more resources at hand.
AI Agents Bring Data-Driven Insights
AI agents can collect and analyze big data in minutes. That makes these intelligent assistants indispensable for business owners making data-driven decisions. Apart from that, you can use AI agents to create reports on market analysis and customer behavior analysis.
AI Agents Give Competitive Advantage
Want to stay one step ahead of your competitors? AI agents ensure you’re always up-to-date with their innovation and marketing capabilities. AI agents aren’t just about speed. They’re changing how companies innovate. Research shows that AI adoption significantly enhances organizational capabilities regarding employee innovation, creativity, and experimentation.
Let Otio be your AI research and writing partner. Try Otio for free today!
Related Reading
• Workflow Examples
• Marketing Automation Workflow
• AI Workflow Automation
• Enterprise Workflow Automation
• AI Web Scraping
• AI That Can Search the Web
• Marketing Automation Workflow Examples
• Types of AI Agents
40 AI Agent Examples for Different Industries
General
1. Otio
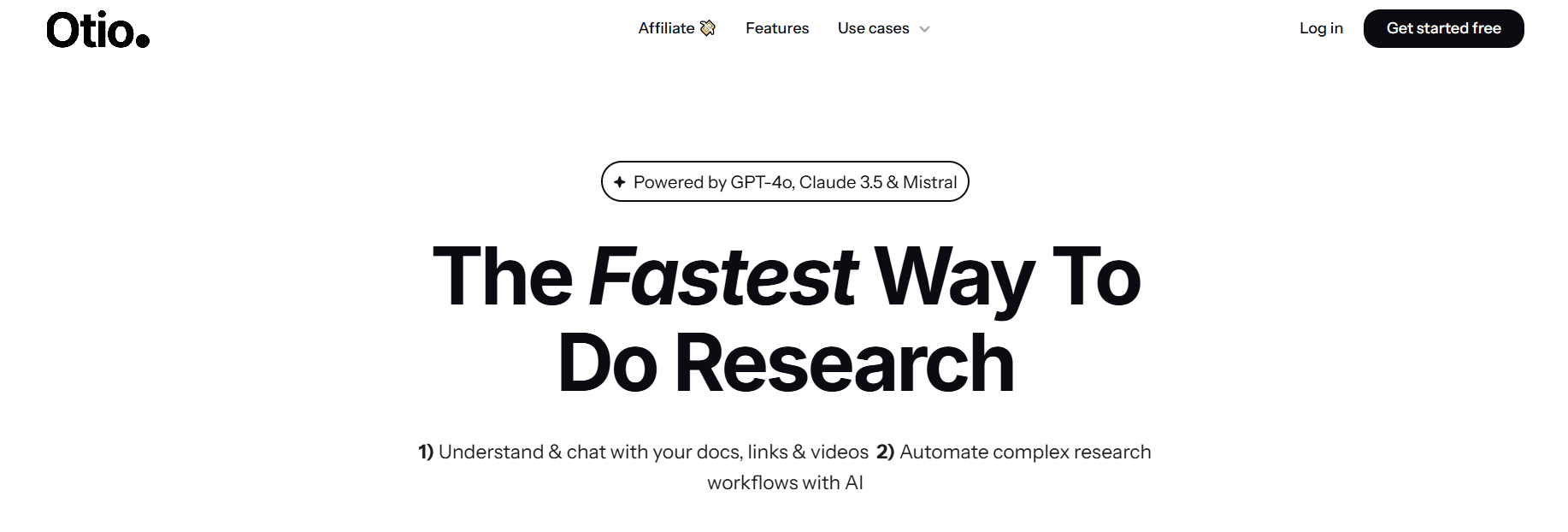
Today, knowledge workers, researchers, and students suffer from content overload and are left to deal with it using fragmented, complex, and manual tooling. Too many settle for stitching together complicated bookmarking, read-it-later, and note-taking apps to get through their workflows. Now that anyone can create content with a button, this problem will only worsen. Otio solves this problem by providing researchers with one AI-native workspace. It helps them:
Collect many data sources, from bookmarks, tweets, and extensive books to YouTube videos.
Extract key takeaways with detailed AI-generated notes and source-grounded Q&A chat.
Create draft outputs using the sources you’ve collected. Otio helps you to go from the reading list to the first draft faster.
Along with this, Otio also helps you write research papers/essays faster. Here are our top features that researchers love: AI-generated notes on all bookmarks (Youtube videos, PDFs, articles, etc.), Otio enables you to chat with individual links or entire knowledge bases, just like you chat with ChatGPT, as well as AI-assisted writing.
Let Otio be your AI research and writing partner—try Otio for free today!
Customer Support
2. ChatGPT (OpenAI)
Businesses widely adopt it to handle customer inquiries in real time. It generates human-like responses, adapts to conversational contexts, and reduces dependency on human agents for repetitive queries.
3. Ada Support
Designed for e-commerce platforms, Ada automates responses to FAQs, streamlining customer interactions while improving response times and accuracy.
4. LivePerson
A conversational AI solution that allows businesses to engage with customers through live chat, voice, and messaging channels. It provides personalized support while integrating with various CRM tools.
5. IBM Watson Assistant
A customizable AI agent that assists enterprises in building chatbots for smooth customer interactions, handling complex queries, and integrating with backend systems.
6. Zendesk AI Bot
Enhances customer service by routing tickets, prioritizing inquiries, and resolving issues faster through AI-powered automation.
Healthcare
7. Babylon Health
Offers AI-powered health diagnostics, combining symptom checkers with virtual consultations to help users better understand their health and access doctors when needed.
8. PathAI
Leverages machine learning to assist pathologists in diagnosing diseases more accurately by analyzing medical images for patterns indicative of cancer and other conditions.
9. Olive
An AI agent for automating routine administrative tasks in hospitals, such as patient registration, insurance claims processing, and billing, to improve operational efficiency.
10. Corti
A real-time decision-support system for emergency medical personnel. It listens to emergency calls and identifies critical symptoms so that it can respond rapidly and accurately.
11. AiCure
Uses computer vision to track patient medication adherence by monitoring if and when pills are taken, improving outcomes in clinical trials and treatments.
Education
12. Duolingo AI
This popular language learning platform employs AI to create personalized lesson plans, adapting to the user’s skill level, strengths, and weaknesses for effective learning.
13. Knewton
An adaptive learning technology that tailors educational content to individual learners, ensuring they focus on areas where they need the most improvement.
14. Socratic (Google)
A homework helper app that uses AI to provide solutions and step-by-step explanations for various subjects, helping students understand challenging topics.
15. Carnegie Learning
A math and literacy program powered by AI to analyze student performance and offer personalized learning pathways to improve outcomes.
16. DreamBox Learning
A K-8 math education platform that adjusts lesson complexity in real time based on student input and understanding.
Finance
17. Kabbage (American Express)
Uses AI to analyze small business financial data and provide quick funding decisions, offering personalized loan options.
18. Betterment
A robo-advisor that helps individuals manage investments by automatically optimizing portfolios based on user-defined goals and risk tolerance.
19. Upstart
An AI-powered lending platform that assesses loan eligibility based on alternative data, such as education and employment history, offering more inclusive credit access.
20. Plaid
It helps businesses securely connect financial accounts to apps, leveraging AI to simplify transaction tracking and user authentication.
21. Clearscore
Provides personalized financial advice by analyzing credit scores and suggesting actionable ways to improve financial health.
Retail & E-commerce
22. Amazon Alexa
An AI-powered virtual assistant that helps users shop online, manage tasks, and control smart devices with voice commands.
23. Shopify AI Chatbot
Assists merchants in managing stores, answering customer queries, and offering personalized product recommendations.
24. Zebra Technologies
Uses AI to optimize supply chain management in retail, ensuring proper inventory levels and streamlining delivery processes.
25. Stylumia
An AI agent for fashion retailers that predicts trends and helps brands optimize their product designs and inventory.
26. Evolv AI
Improves website and app performance by running continuous experiments to understand user behavior and increase conversions.
Transportation
27. Tesla Autopilot
An AI-driven advanced driver assistance system (ADAS) that enables semi-autonomous driving by using cameras, sensors, and real-time decision-making.
Waymo: A self-driving technology company leveraging AI to navigate urban environments, aiming for fully autonomous transportation solutions.
28. Uber AI
Improves ride-matching, route optimization, and pricing strategies using machine learning to enhance the rider and driver experience.
29. Lilium AI
Aerial transportation company using AI for piloting electric vertical takeoff and landing (eVTOL) jets for urban air mobility.
30. HERE Technologies
Provides AI-powered mapping solutions for autonomous vehicles, offering real-time traffic and road conditions data.
Entertainment
31. Netflix Recommendation Engine
Uses AI to analyze viewer preferences and behavior, delivering personalized content recommendations to enhance user engagement.
32. Spotify AI DJ
Offers personalized playlists and mood-based music recommendations by understanding user preferences through machine learning.
33. YouTube AI
Optimizes video recommendations by analyzing user activity and content preferences, increasing watch time and satisfaction.
34. Runway AI
Helps creators edit videos and images with AI tools for advanced effects, automatic color grading, and object removal.
35. DeepMind MuZero
Demonstrates cutting-edge AI by mastering board and video games, showcasing complex decision-making capabilities.
Manufacturing
36. Siemens MindSphere
An industrial IoT solution using AI to analyze production line data, optimize processes, and predict equipment failures.
37. GE Brilliant Factory
Combines AI and IoT to improve factory efficiency by identifying bottlenecks and optimizing production workflows.
38. Bright Machines
Uses AI-driven micro factories to automate electronics manufacturing, offering speed and precision for high-demand products.
39. NVIDIA Isaac
A robotics platform that enables AI-driven robots to automate complex manufacturing tasks, such as assembly and quality inspection.
40. Fero Labs
AI software that helps manufacturers improve quality and reduce waste by analyzing production data and suggesting process adjustments."
7 Types of AI Agents To Help Your Business
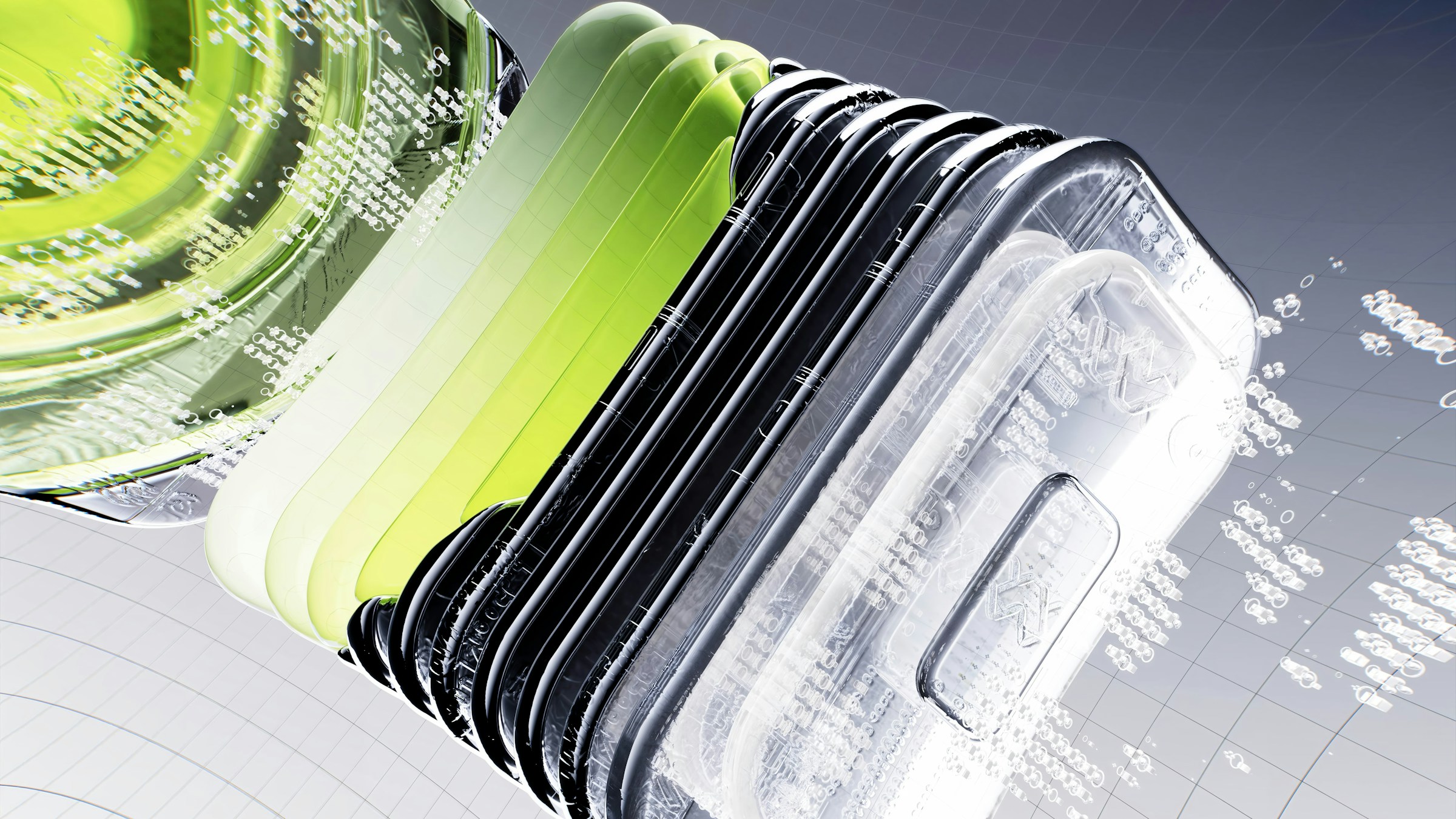
1. Simple Reflex Agents
Simple reflex agents represent one of the most fundamental types of artificial intelligence. These agents base their decisions on current sensory input, immediately responding to environmental stimuli without needing memory or learning processes. Their behavior follows predefined condition-action rules that detail how to react to particular inputs. Due to their complexity limitations, simple reflex agents are very efficient and easy to implement, especially in environments where the range of possible actions is narrow.
Key Components
Sensors: Similar to human senses, sensors gather information from the environment. These are essential input devices for a simple reflex agent that detects specific environmental conditions such as temperature, light, or motion.
Condition-action rules: These predefined rules determine how the agent responds to specific inputs. The logic is direct. If the agent detects a particular condition, it immediately performs a corresponding action.
Actuators: These execute decisions made by the agent, translating them into physical or digital responses that alter the environment in some way, such as activating a heating system or turning on lights.
Use Cases
Simple reflex agents work well in transparent, predictable environments with limited variables. Examples include:
Industrial safety sensors that immediately shut down machinery when detecting an obstruction in the work area.
Automated sprinkler systems that activate based on smoke detection.
Email auto-responders that send predefined messages based on specific keywords or sender addresses.
2. Model-Based Reflex Agents
Model-based reflex agents are more sophisticated intelligent agents designed to operate in partially observable environments. Unlike simple reflex agents, which react solely based on current sensory information, model-based agents maintain an internal representation of the world. This model tracks how the environment evolves, allowing the agent to infer unobserved aspects of the current state. While these agents do not actually “remember” past states like more advanced agents do, they use their world model to make better decisions about the current state.
Key Components
State Tracker: Maintains information about the current state of the environment based on the world model and sensor history.
World Model: Contains two key types of knowledge: how the environment evolves independent of the agent and how the agent’s actions affect the environment.
Reasoning Component: Uses the world model and current state to determine appropriate actions based on condition-action rules.
Use Cases
These agents are suitable for environments where the current state isn’t fully observable from sensor data alone.
Examples Include
Smart home security systems: Using models of standard household activity patterns to distinguish between routine events and potential security threats.
Quality control systems: Monitoring manufacturing processes by maintaining a model of normal operations to detect deviations.
Network monitoring tools: Tracking network state and traffic patterns to identify potential issues or anomalies.
3. Goal-Based Agents
Goal-based agents are designed to pursue specific objectives by considering the future consequences of their actions. Unlike reflex agents that act based on rules or world models, goal-based agents plan sequences of actions to achieve desired outcomes. They use search and planning algorithms to find action sequences that lead to their goals.
Key Components
Goal State: A clear description of what the agent aims to achieve.
Planning Mechanism: The ability to search through possible sequences of actions that could lead to the goal.
State Evaluation: Methods to assess whether potential future states move closer to or further from the goal.
Action Selection: Choosing actions based on their predicted contribution toward reaching the goal.
World Model: Understanding how actions change the environment, used for planning.
Use Cases
Goal-based agents are suited for tasks with clear objectives and predictable action outcomes.
Examples Include
Industrial robots: Following specific sequences to assemble products.
Automated warehouse systems: Planning optimal paths to retrieve items.
Intelligent heating systems: Planning temperature adjustments to reach desired comfort levels efficiently.
Inventory management systems: Planning reorder schedules to maintain target stock levels.
Task scheduling systems: Organizing sequences of operations to meet completion deadlines.
4. Learning Agents
A learning agent is an artificial intelligence system capable of improving its behavior over time by interacting with its environment and learning from its experiences. These agents modify their behavior based on feedback and experience, using various learning mechanisms to optimize performance. Unlike more straightforward agent types, they can discover how to achieve their goals through experience rather than purely relying on pre-programmed knowledge.
Key Components
Performance Element: The component that selects external actions, similar to the decision-making modules in more straightforward agents.
Critic: Provides feedback on the agent’s performance by evaluating outcomes against standards, often using a reward or performance metric.
Learning Element: Uses critic’s feedback to improve the performance element, determining how to modify behavior to do better in the future.
Problem Generator: Suggests exploratory actions that lead to new experiences and better future decisions.
Use Cases
Learning agents are suited for environments where optimal behavior isn’t known in advance and must be learned through experience.
Examples Include
Industrial process control: Learning optimal settings for manufacturing processes through trial and error.
Energy management systems: Learning patterns of usage to optimize resource consumption.
Customer service chatbots: Improving response accuracy based on interaction outcomes.
Quality control systems: Learning to identify defects more accurately over time.
5. Utility-Based Agents
A utility-based agent makes decisions by evaluating the potential outcomes of its actions and choosing the one that maximizes overall utility. Unlike goal-based agents that aim for specific states, utility-based agents can handle tradeoffs between competing goals by assigning numerical values to different outcomes.
Key Components
Utility Function: A mathematical function that maps states to numerical values, representing the desirability of each state.
State Evaluation: Methods to assess current and potential future states regarding their utility.
Decision Mechanism: Processes for selecting actions that are expected to maximize utility.
Environment Model: Understanding of how actions affect the environment and resulting utilities.
Use Cases
Utility-based agents are suited for scenarios requiring a balance between multiple competing objectives.
Examples Include
Resource allocation systems: Balancing machine usage, energy consumption, and production goals.
Innovative building management: Optimizing between comfort, energy efficiency, and maintenance costs.
Scheduling systems: Balancing task priorities, deadlines, and resource constraints.
6. Hierarchical Agents
Hierarchical agents are structured in a tiered system, where higher-level agents manage and direct the actions of lower-level agents. This architecture breaks down complex tasks into manageable subtasks, allowing for more organized control and decision-making.
Key Components
Task Decomposition: This breaks down complex tasks into simpler subtasks that lower-level agents can manage.
Command Hierarchy: Defines how control and information flow between different levels of agents.
Coordination Mechanisms: Ensures different levels of agents work together coherently.
Goal Delegation: Translates high-level objectives into specific tasks for lower-level agents.
Use Cases
Hierarchical agents are best suited for systems with clear task hierarchies and well-defined subtasks.
Examples Include
Manufacturing control systems: Coordinating different stages of production processes.
Building automation: Managing basic systems like HVAC and lighting through layered control.
Robotic task planning: Breaking down simple robotic tasks into basic movements and actions.
7. Multi-Agent Systems
A multi-agent system involves multiple autonomous agents interacting within a shared environment, working independently or cooperatively to achieve individual or collective goals. While often confused with more advanced AI systems, traditional MAS focuses on simple agents interacting through basic protocols and rules.
Types of Multi-Agent Systems
Cooperative Systems: Agents share information and resources to achieve common goals. For example, multiple robots work together on basic assembly tasks.
Competitive Systems: Agents compete for resources following defined rules, like multiple bidding agents in a simple auction system.
Mixed Systems: Combines both cooperative and competitive behaviors, such as agents sharing some information while competing for limited resources.
Key Components
Communication Protocols: Define how agents exchange information.
Interaction Rules: Specify how agents can interact and what actions are permitted.
Resource Management: Methods for handling shared resources between agents.
Coordination Mechanisms: Systems for organizing agent activities and preventing conflicts.
Use Cases
Multi-agent systems best suit scenarios with clear interaction rules and simple agent behaviors.
Examples Include
Warehouse management: Multiple robots coordinate to move and sort items.
Basic manufacturing: Coordinating simple assembly tasks between multiple machines.
Resource allocation: Managing shared resources like processing time or storage space.
Related Reading
• AI Business Automation
• How to Automate Tasks
• How to Build Workflows
• Workflow Automation Tools
• Document Workflow Automation
• AI Productivity Tools
• Workflow Apps
• How to Use AI to Automate Tasks
• AI Workflows
• CRM Workflow Automation
• AI Automation Tools
Supercharge Your Researching Ability With Otio — Try Otio for Free Today
AI agents can help students sift through the noise of academic research. Otio is an AI research assistant who helps students collect, organize, and create writing outputs for scholarly research.
Let Otio be your AI research and writing partner—try Otio for free today!
Related Reading
• HR Workflow Automation
• Best AI Agents
• Workflow Automation for Small Business
• Marketing Workflow Automation
• Low Code Workflow Automation
• Sales Workflow Automation
• AI Workflow Tools
• AI Financial Statement Analysis
• AI Tools for Finance